Decreased cortical thickness and normal regional homogeneity underlying cognitive impairment in cerebral small vessel disease
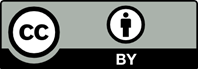
Cerebral small vessel disease (CSVD) is the most common cause of vascular cognitive impairment (CI). However, the cognitive performance of individuals with CSVD varies considerably. Early identification of neuroimaging changes related to CI caused by CSVD is important for the prediction and management of CI. The present study aimed to explore the early alterations in cortical thickness and regional homogeneity (ReHo) related to CI in people with CSVD. A total of 106 participants with CSVD with CI, 77 participants with CSVD without CI, and 121 control participants underwent neuropsychological tests and multimodal magnetic resonance imaging scans. Cortical thickness was analyzed using FreeSurfer software, and ReHo patterns were explored using the DPARSF toolbox in brain regions that exhibited alterations in cortical thickness. The CSVD with CI group exhibited decreased cortical thickness but normal ReHo in the right anterior cingulate gyrus (ACG), right cuneus, bilateral insula, and right middle temporal gyrus compared with that in the other two groups. Specifically, both cortical thickness and ReHo in the right ACG were significantly associated with memory performance in CSVD patients with CI. In addition, impaired visuospatial function occurred earlier than the decline of global function in CSVD patients and was related to cortical thinning in the right middle temporal gyrus. In conclusion, decreased cortical thickness but normal ReHo in the right ACG, right cuneus, bilateral insula, and right middle temporal gyrus are characteristic alterations related to the development of CI in CSVD patients. These findings may contribute to the early prediction of CI in CSVD patients.
Huang L, Chen X, Sun W, et al., 2020, Early segmental white matter fascicle microstructural damage predicts the corresponding cognitive domain impairment in cerebral small vessel disease patients by automated fiber quantification. Front Aging Neurosci, 12: 598242. https://doi.org/10.3389/fnagi.2020.598242
Cannistraro RJ, Badi M, Eidelman BH, et al., 2019, CNS small vessel disease: A clinical review. Neurology, 92(24): 1146–1156.
Jokinen H, Melkas S, Madureira S, et al., 2016, Cognitive reserve moderates long-term cognitive and functional outcome in cerebral small vessel disease. J Neurol Neurosurg Psychiatry, 87: 1296–1302. https://doi.org/10.1136/jnnp-2016-313914
Liu R, Wu W, Ye Q, et al., 2019, Distinctive and pervasive alterations of functional brain networks in cerebral small vessel disease with and without cognitive impairment. Dement Geriatr Cogn Disord, 47: 55–67. https://doi.org/10.1159/000496455
Chen H, Zhu H, Huang L, et al., 2022, The flexibility of cognitive reserve in regulating the frontoparietal control network and cognitive function in subjects with white matter hyperintensities. Behav Brain Res, 2022: 113831. https://doi.org/10.1016/j.bbr.2022.113831
Dey AK, Stamenova V, Turner G, et al., 2016, Pathoconnectomics of cognitive impairment in small vessel disease: A systematic review. Alzheimers Dement, 12: 831–845. https://doi.org/10.1016/j.jalz.2016.01.007
Chen H, Huang L, Yang D, et al., 2019, Nodal global efficiency in front-parietal lobe mediated periventricular white matter hyperintensity (PWMH)-related cognitive impairment. Front Aging Neurosci, 11: 347. https://doi.org/10.3389/fnagi.2019.00347
Banerjee G, Wilson D, Jager HR, et al., 2016, Novel imaging techniques in cerebral small vessel diseases and vascular cognitive impairment. Biochim Biophys Acta, 1862: 926–938. https://doi.org/10.1016/j.bbadis.2015.12.010
Lambert C, Narean JS, Benjamin P, et al., 2015, Characterising the grey matter correlates of leukoaraiosis in cerebral small vessel disease. Neuroimage Clin, 9: 194–205. https://doi.org/10.1016/j.nicl.2015.07.002
Li H, Jia X, Li Y, et al., 2021, Aberrant amplitude of low-frequency fluctuation and degree centrality within the default mode network in patients with vascular mild cognitive impairment. Brain Sci, 11: 1534. https://doi.org/10.3390/brainsci11111534
Wang Y, Yang Y, Wang T, et al., 2020, Correlation between white matter hyperintensities related gray matter volume and cognition in cerebral small vessel disease. J Stroke Cerebrovasc Dis, 29: 105275. https://doi.org/10.1016/j.jstrokecerebrovasdis.2020.105275
Tang J, Shi L, Zhao Q, et al., 2017, Coexisting cortical atrophy plays a crucial role in cognitive impairment in moderate to severe cerebral small vessel disease patients. Discov Med, 23: 175–182.
Zang Y, Jiang T, Lu Y, et al., 2004, Regional homogeneity approach to fMRI data analysis. Neuroimage, 22: 394–400. https://doi.org/10.1016/j.neuroimage.2003.12.030
Ye Q, Chen X, Qin R, et al., 2019, Enhanced regional homogeneity and functional connectivity in subjects with white matter hyperintensities and cognitive impairment. Front Neurosci, 13: 695. https://doi.org/10.3389/fnins.2019.00695
Prins ND, Scheltens P. White matter hyperintensities, cognitive impairment and dementia: An update. Nat Rev Neurol, 11: 157–165. https://doi.org/10.1038/nrneurol.2015.10
Wardlaw JM, Smith EE, Biessels GJ, et al., 2013, Neuroimaging standards for research into small vessel disease and its contribution to ageing and neurodegeneration. Lancet Neurol, 12:822–838. https://doi.org/10.1016/S1474-4422(13)70124-8
Lawrence AJ, Chung AW, Morris RG, et al., 2014, Structural network efficiency is associated with cognitive impairment in small-vessel disease. Neurology, 83: 304–311. https://doi.org/10.1212/WNL.0000000000000612
Lu J, Li D, Li F, et al., 2011, Montreal cognitive assessment in detecting cognitive impairment in Chinese elderly individuals: A population-based study. J Geriatr Psychiatry Neurol, 24: 184–190. https://doi.org/10.1177/0891988711422528
Fischl B, 2012, FreeSurfer. Neuroimage, 62: 774–781. https://doi.org/10.1016/j.neuroimage.2012.01.021
Sled JG, Zijdenbos AP, Evans AC, 1998, A nonparametric method for automatic correction of intensity nonuniformity in MRI data. IEEE Trans Med Imaging, 17: 87–97. https://doi.org/10.1109/42.668698
Dale AM, Fischl B, Sereno MI, 1999, Cortical surface-based analysis. I. Segmentation and surface reconstruction. Neuroimage, 9: 179–194. https://doi.org/10.1006/nimg.1998.0395
Fischl B, Liu A, Dale AM, 2011, Automated manifold surgery: Constructing geometrically accurate and topologically correct models of the human cerebral cortex. IEEE Trans Med Imaging, 20: 70–80. https://doi.org/10.1109/42.906426
Fischl B, Sereno MI, Dale AM, 1999, Cortical surface-based analysis. II: Inflation, flattening, and a surface-based coordinate system. Neuroimage, 9(2): 195–207. https://doi.org/10.1006/nimg.1998.0396
Fischl B, Sereno MI, Tootell RB, et al., 1999, High-resolution intersubject averaging and a coordinate system for the cortical surface. Hum Brain Mapp, 8: 272–284. https://doi.org/10.1002/(sici)1097-0193(1999)8:4<272::aid-hbm10>3.0.co;2-4
Fischl B, van der Kouwe A, Destrieux C, et al., 2004, Automatically parcellating the human cerebral cortex. Cereb Cortex, 14: 11–22. https://doi.org/10.1093/cercor/bhg087
Desikan RS, Segonne F, Fischl B, et al., 2006, An automated labeling system for subdividing the human cerebral cortex on MRI scans into gyral based regions of interest. Neuroimage, 31: 968–980. https://doi.org/10.1016/j.neuroimage.2006.01.021
Fischl B, Dale AM, 2000, Measuring the thickness of the human cerebral cortex from magnetic resonance images. Proc Natl Acad Sci U S A, 97: 11050–11055. https://doi.org/10.1073/pnas.200033797
Klein A, Tourville J, 2012, 101 labeled brain images and a consistent human cortical labeling protocol. Front Neurosci, 6: 171. https://doi.org/10.3389/fnins.2012.00171
Kloppenborg RP, Nederkoorn PJ, Geerlings MI, et al., 2014, Presence and progression of white matter hyperintensities and cognition: A meta-analysis. Neurology, 82: 2127–38. https://doi.org/10.1212/WNL.0000000000000505
Alber J, Alladi S, Bae HJ, et al., 2019, White matter hyperintensities in vascular contributions to cognitive impairment and dementia (VCID): Knowledge gaps and opportunities. Alzheimers Dement (NY) 5: 107–117. https://doi.org/10.1016/j.trci.2019.02.001
Brundel M, Kwa VI, Bouvy WH, et al., 2014, Cerebral microbleeds are not associated with long-term cognitive outcome in patients with transient ischemic attack or minor stroke. Cerebrovasc Dis, 37: 195–202. https://doi.org/10.1159/000358119
Doi H, Inamizu S, Saito BY, et al., 2015, Analysis of cerebral lobar microbleeds and a decreased cerebral blood flow in a memory clinic setting. Intern Med, 54: 1027–1033. https://doi.org/10.2169/internalmedicine.54.3747
Makin SD, Turpin S, Dennis MS, et al., 2013, Cognitive impairment after lacunar stroke: Systematic review and meta-analysis of incidence, prevalence and comparison with other stroke subtypes. J Neurol Neurosurg Psychiatry, 84: 893–900. https://doi.org/10.1136/jnnp-2012-303645
Chen L, Song J, Cheng R, et al., 2020, Cortical thinning in the medial temporal lobe and precuneus is related to cognitive deficits in patients with subcortical ischemic vascular disease. Front Aging Neurosci, 12: 614833. https://doi.org/10.3389/fnagi.2020.614833
Ni L, Liu R, Yin Z, et al., 2016, Aberrant spontaneous brain activity in patients with mild cognitive impairment and concomitant lacunar infarction: A resting-state functional MRI study. J Alzheimers Dis, 50: 1243–1254. https://doi.org/10.3233/JAD-150622
Gasquoine PG, 2013, Localization of function in anterior cingulate cortex: from psychosurgery to functional neuroimaging. Neurosci Biobehav Rev, 37: 340–348. https://doi.org/10.1016/j.neubiorev.2013.01.002
Chen Y, Wang J, Zhang J, et al., 2014, Aberrant functional networks connectivity and structural atrophy in silent lacunar infarcts: Relationship with cognitive impairments. J Alzheimers Dis, 42: 841–850. https://doi.org/10.3233/JAD-140948
Cabeza R, Nyberg L, 2000, Imaging cognition II: An empirical review of 275 PET and fMRI studies. J Cogn Neurosci, 12: 1–47. https://doi.org/10.1162/08989290051137585
Kobayashi Y, Morizumi T, Nagamatsu K, et al., 2021, Persistent working memory impairment associated with cerebral infarction in the anterior cingulate cortex: A case report and a literature review. Intern Med, 60: 3473–3476. https://doi.org/10.2169/internalmedicine.6927-20
Abd Razak MA, Ahmad NA, Chan YY, et al., 2019, Validity of screening tools for dementia and mild cognitive impairment among the elderly in primary health care: A systematic review. Public Health, 169: 84–92. https://doi.org/10.1016/j.puhe.2019.01.001
Zhuang L, Yang Y, Gao J, 2021, Cognitive assessment tools for mild cognitive impairment screening. J Neurol, 268: 1615–1622. https://doi.org/10.1007/s00415-019-09506-7
Tallarita GM, Parente A, Giovagnoli AR, 2019, The visuospatial pattern of temporal lobe epilepsy. Epilepsy Behav, 101: 106582. https://doi.org/10.1016/j.yebeh.2019.106582
Nie S, Shen C, Guo Y, et al., 2021, Preliminary findings on visual event-related potential P3 in asymptomatic patients with cerebral small vessel disease. Neuropsychiatr Dis Treat, 17: 3379–3394. https://doi.org/10.2147/NDT.S338717
Farivar R, 2009, Dorsal-ventral integration in object recognition. Brain Res Rev, 61:144–153. https://doi.org/10.1016/j.brainresrev.2009.05.006
Uithol S, Bryant KL, Toni I, et al., 2021, The anticipatory and task-driven nature of visual perception. Cereb Cortex, 31: 5354–5362.
Ibarretxe-Bilbao N, Junque C, Marti MJ, et al., 2011, Brain structural MRI correlates of cognitive dysfunctions in Parkinson’s disease. J Neurol Sci, 310: 70–74. https://doi.org/10.1016/j.jns.2011.07.054
Andrade K, Kas A, Valabrègue R, et al., 2012, Visuospatial deficits in posterior cortical atrophy: Structural and functional correlates. J Neurol Neurosurg Psychiatry, 83: 860–863. https://doi.org/10.1136/jnnp-2012-302278
Schulz M, Malherbe C, Cheng B, et al., 2021, Functional connectivity changes in cerebral small vessel disease a systematic review of the resting-state MRI literature. BMC Med, 19: 103. https://doi.org/10.1186/s12916-021-01962-1