Domain expert evaluation of advanced visual computing solutions and 3D printing for the planning of the left atrial appendage occluder interventions
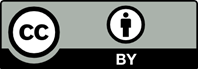
Advanced visual computing solutions and three-dimensional (3D) printing are moving from engineering to clinical pipelines for training, planning, and guidance of complex interventions. 3D imaging and rendering, virtual reality (VR), and in-silico simulations, as well as 3D printing technologies provide complementary information to better understand the structure and function of the organs, thereby improving and personalizing clinical decisions. In this study, we evaluated several advanced visual computing solutions, such as web-based 3D imaging visualization, VR, and computational fluid simulations, together with 3D printing, for the planning of the left atrial appendage occluder (LAAO) device implantations. Six cardiologists tested different technologies in pre-operative data of five patients to identify the usability, limitations, and requirements for the clinical translation of each technology through a qualitative questionnaire. The obtained results demonstrate the potential impact of advanced visual computing solutions and 3D printing to improve the planning of LAAO interventions as well as the need for their integration into a single workflow to be used in a clinical environment.
1. Kikinis R, Pieper SD, Vosburgh KG, 2014, 3D slicer: A platform for subject-specific image analysis, visualization, and clinical support. In: jolesz fa, editor. Intraoperative imaging and image-guided therapy. New York: springer, p277–289.
2. Wang DD, Qian Z, Vukicevic M, et al., 2021, 3D printing, computational modeling, and artificial intelligence for structural heart disease. JACC Cardiovasc Imaging, 14: 41–60. https://doi.org/10.1016/j.jcmg.2019.12.022
3. Wang DD, Geske J, Choi AD, et al., 2018, Navigating a career in structural heart disease interventional imaging. JACC Cardiovasc Imaging, 11: 1928–1930. https://doi.org/10.1016/j.jcmg.2018.07.010
4. Salavitabar A, Figueroa CA, Lu JC, et al., 2020, Emerging 3D technologies and applications within congenital heart disease: teach, predict, plan and guide. Future Cardiol, 16: 695–709. https://doi.org/10.2217/fca-2020-0004
5. Goo HW, Park SJ, Yoo SJ, 2020, Advanced medical use of three-dimensional imaging in congenital heart disease: Augmented reality, mixed reality, virtual reality, and three dimensional printing. Korean J Radiol, 21:133–145. https://doi.org/10.3348/kjr.2019.0625
6. Kohli K, Wei ZA, Sadri V, et al., 2020, Framework for planning TMVR using 3-D imaging, in silico modeling, and virtual reality. Struct Hear, 4: 336–341. https://doi.org/10.1080/24748706.2020.1762268
7. Devgun J, De Potter T, Fabbricatore D, et al., 2022, Precath laboratory planning for left atrial appendage occlusion optional or essential? Interv Cardiol Clin, 11: 143–152. https://doi.org/10.1016/j.iccl.2021.11.003
8. Vivoli G, Gasparotti E, Rezzaghi M, et al., 2019, Simultaneous functional and morphological assessment of left atrial appendage by 3D virtual models. J Healthc Eng, 2019: 7095845.
https://doi.org/10.1155/2019/7095845
9. Saw J, Fahmy P, Spencer R, et al., 2016, Comparing measurements of ct angiography, tee, and fluoroscopy of the left atrial appendage for percutaneous closure. J Cardiovasc Electrophysiol, 27: 414–422. https://doi.org/10.1111/jce.12909
10. Hascoet S, Smolka G, Bagate F, et al., 2018, Multimodality imaging guidance for percutaneous paravalvular leak closure: Insights from the multi-centre ffpp register. Arch Cardiovasc Dis, 111: 421–431. https://doi.org/10.1016/j.acvd.2018.05.001
11. Karagodin I, Addetia K, Singh A, et al., 2020, Improved delineation of cardiac pathology using a novel three dimensional echocardiographic tissue transparency tool. J Am Soc Echocardiogr, 33: 1316–1323. https://doi.org/10.1016/j.echo.2020.08.005
12. Aguado AM, Olivares AL, Yagüe C, et al., 2019, In silico optimization of left atrial appendage occluder implantation using interactive and modeling Tools. Front Physiol, 10: 237. https://doi.org/10.3389/fphys.2019.00237
13. Vukicevic M, Mosadegh B, Min JK, et al., 2017, Cardiac 3D printing and its future directions. JACC Cardiovasc Imaging, 10: 171–184. https://doi.org/10.1016/j.jcmg.2016.12.001
14. Forte MNV, Hussain T, Roest A, et al., 2019, Living the heart in three dimensions: applications of 3D printing in CHD. Cardiol Young, 29: 733–743. https://doi.org/10.1017/S1047951119000398
15. Fan Y, Yang F, Cheung GSH, et al., 2019, Device sizing guided by echocardiography-based three-dimensional printing is associated with superior outcome after percutaneous left atrial appendage occlusion. J Am Soc Echocardiogr, 32: 708–719.e1. https://doi.org/10.1016/j.echo.2019.02.003
16. Wang DD, Gheewala N, Shah R, et al., 2018, Three dimensional printing for planning of structural heart interventions. Interv Cardiol Clin, 7: 415–423. https://doi.org/10.1016/j.iccl.2018.04.004
17. Eng MH, Wang DD, 2018, Computed tomography for left atrial appendage occlusion case planning. Interv Cardiol Clin, 7: 367–378. https://doi.org/10.1016/j.iccl.2018.03.003
18. Kim WD, Cho I, Kim YD, et al., 2022., Improving left atrial appendage occlusion device size determination by three dimensional printing-based preprocedural simulation. Front Cardiovasc Med, 9: 830062. https://doi.org/10.3389/fcvm.2022.830062
19. Conti M, Marconi S, Muscogiuri G, et al., 2019, Left atrial appendage closure guided by 3d computed tomography printing technology: a case control study. J Cardiovasc Comput Tomogr, 13: 336–339. https://doi.org/10.1016/j.jcct.2018.10.024
20. Mendez A, Hussain T, Hosseinpour AR, et al., 2019, Virtual reality for preoperative planning in large ventricular septal defects. Eur Heart J, 40: 1092. https://doi.org/10.1093/eurheartj/ehy685
21. Tandon A, Burkhardt BEU, Batsis M, et al., 2019, Sinus venosus defects. JACC Cardiovasc Imaging, 12: 921–924. https://doi.org/10.1016/j.jcmg.2018.10.013
22. Southworth MK, Silva JR, Silva JN, 2020, Use of extended realities in cardiology. Trends Cardiovasc Med, 30: 143–148. https://doi.org/10.1016/j.tcm.2019.04.005
23. Nam HH, Herz C, Lasso A, et al., 2020, Simulation of transcatheter atrial and ventricular septal defect device closure within three-dimensional echo cardiography derived heart models on screen and in virtual reality. J Am Soc Echocardiogr, 33: 641–644.e2. https://doi.org/10.1016/j.echo.2020.01.011
24. Narang A, Hitschrich N, Mor-Avi V, et al., 2020, Virtual reality analysis of three-dimensional echocardiographic and cardiac computed tomographic data sets. J Am Soc Echocardiogr, 33: 1306–1315. https://doi.org/10.1016/j.echo.2020.06.018
25. Sanon S, Lim DS, 2019, Update on left atrial appendage occlusion an overview of innovative preprocedural and intraprocedural imaging techniques, trial data, and current laao devices and devices in development. Struct Heart Dis, 13: 35–41
26. Lindner S, Behnes M, Wenke A, et al., 2019, Assessment of peri-device leaks after interventional left atrial appendage closure using standardized imaging by cardiac computed tomography angiography. Int J Cardiovasc Imaging, 35: 725–731. https://doi.org/10.1007/s10554-018-1493-z
27. Medina E, Aguado AM, Mill J, et al., 2020, VRIDAA: Virtual reality platform for training and planning implantations of occluder devices in left atrial appendages. Eurographics Work Vis Comput Biol Med, 31–35. https://doi.org/10.2312/vcbm.20201168
28. Ribeiro JM, Astudillo P, de Backer O, et al., 2022, Artificial intelligence and transcatheter interventions for structural heart disease: A glance at the (near) future, Trends Cardiovasc Med, 32:153-159. https://doi.org/10.1016/j.tcm.2021.02.002
29. Corral-Acero J, Margara F, Marciniak M, et al., 2020, The “Digital Twin” to enable the vision of precision cardiology. Eur Heart J, 41: 4556–4564. https://doi.org/10.1093/eurheartj/ehaa159
30. Garot P, Iriart X, Aminian A, et al., 2020, Value of feops heart guide patient-specific computational simulations in the planning of left atrial appendage closure with the amplatzer amulet closure device: Rationale and design of the predictlaa study. Open Hear, 7: e001326. https://doi.org/10.1136/openhrt-2020-001326
31. Naci H, Salcher-Konrad M, Mcguire A, et al., 2019, Impact of predictive medicine on therapeutic decision making: A randomized controlled trial in congenital heart disease. NPJ Digit Med, 2: 17. https://doi.org/10.1038/s41746-019-0085-1
32. Otani T, Al-Issa A, Pourmorteza A, et al., 2016, A computational framework for personalized blood flow analysis in the human left atrium. Ann Biomed Eng, 44: 3284–3294. https://doi.org/10.1007/s10439-016-1590-x
33. Fanni BM, Capellini K, Di Leonardo M, et al., 2020, Correlation between laa morphological features and computational fluid dynamics analysis for non-valvular atrial fibrillation patients. Appl Sci, 10: 1448. https://doi.org/10.3390/app10041448
34. Pons MI, Mill J, Fernandez-Quilez A, et al., 2022, Joint analysis of morphological parameters and in silico haemodynamics of the left atrial appendage for thrombogenic risk assessment. J Interv Cardiol, 2022: 9125224. https://doi.org/10.1155/2022/9125224
35. Feng L, Gao H, Griffith B, et al., 2019, Analysis of a coupled fluid-structure interaction model of the left atrium and mitral valve. Int J Numer Method Biomed Eng, 35: e3254. https://doi.org/10.1002/cnm.3254
36. Mill J, Olivares AL, Arzamendi D, et al., 2020, Impact of flow-dynamics on device related thrombosis after left atrial appendage occlusion. Can J Cardiol, 36: 968.e13–968.e14. https://doi.org/10.1016/j.cjca.2019.12.036
37. Mill J, Agudelo V, Li CH, et al., 2021, Patient-specific flow simulation analysis to predict device-related thrombosis in left atrial appendage occluders. REC Interv Cardiol, 3: 278–285.
https://doi.org/10.24875/RECICE.M21000224
38. Brooke J, 1996, SUS-A quick and dirty usability scale. In: Usability Evaluation in Industry. Vol. 189, Boca Raton, Florida: CRC Press, p4–7.
39. Mill J, Agudelo V, Olivares AL, et al., 2021, Sensitivity analysis of in silico fluid simulations to predict thrombus formation after left atrial appendage occlusion. Mathematics, 9: 2304. https://doi.org/10.3390/math9182304
40. Bangor A, Kortum P, Miller J, 2009, Determining what individual sus scores mean; Adding an adjective rating. J Usability Stud, 4: 114–123.
41. Viceconti M, Pappalardo F, Rodriguez B, et al., 2021, In silico trials: Verification, validation and uncertainty quantification of predictive models used in the regulatory evaluation of biomedical products. Methods, 185: 120–127. https://doi.org/10.1016/j.ymeth.2020.01.011
42. Zaccaria A, Danielli F, Gasparotti E, et al., 2020, Left atrial appendage occlusion device: development and validation of a finite element model. Med Eng Phys, 82: 104–108. https://doi.org/10.1016/j.medengphy.2020.05.019
43. Celi S, Gasparotti E, Capellini K, et al., 2021, 3D printing in modern cardiology. Curr Pharm Des, 27: 1918–1930. https://doi.org/10.2174/1381612826666200622132440