Deep Learning-Assisted Nephrotoxicity Testing with Bioprinted Renal Spheroids
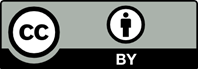
We used arrays of bioprinted renal epithelial cell spheroids for toxicity testing with cisplatin. The concentrationdependent cell death rate was determined using a lactate dehydrogenase assay. Bioprinted spheroids showed enhanced sensitivity to the treatment in comparison to monolayers of the same cell type. The measured dose-response curves revealed an inhibitory concentration of the spheroids of IC50 = 9 ± 3 µM in contrast to the monolayers with IC50 = 17 ± 2 µM. Fluorescent labeling of a nephrotoxicity biomarker, kidney injury molecule 1 indicated an accumulation of the molecule in the central lumen of the spheroids. Finally, we tested an approach for an automatic readout of toxicity based on microscopic images with deep learning. Therefore, we created a dataset comprising images of single spheroids, with corresponding labels of the determined cell death rates for training. The algorithm was able to distinguish between three classes of no, mild, and severe treatment effects with a balanced accuracy of 78.7%.
1. Breslin S, O’Driscoll L, 2013, Three-dimensional Cell Culture: The Missing Link in Drug Discovery. Drug Discov Today, 18:240. https://doi.org/10.1016/j.drudis.2012.10.003
2. Homan KA, Kolesky DB, Skylar-Scott MA, et al., 2016. Bioprinting of 3D Convoluted Renal Proximal Tubules on Perfusable Chips. Sci Rep, 6:34845. https://doi.org/10.1038/srep34845
3. Secker PE, Luks L, Schlichenmaier N, et al., 2018, RPTEC/TERT1 Cells form Highly Differentiated Tubules when Cultured in a 3D Matrix. ALTEX, 35:223. https://doi.org/10.14573/altex.1710181
4. Kaminski MM, Tosic J, Kresbach C, et al., 2016. Direct Reprogramming of Fibroblasts into Renal Tubular Epithelial Cells by Defined Transcription Factors. Nat Cell Biol, 18:1269. https://doi.org/10.1038/ncb3437
5. Buzhor E, Harari-Steinberg O, Omer D, et al., 2011, Kidney Spheroids Recapitulate Tubular Organoids Leading to Enhanced Tubulogenic Potency of Human Kidney-derived Cells. Tissue Eng Part A, 17:2305. https://doi.org/10.1089/ten.TEA.2010.0595
6. Mota C, Camarero-Espinosa S, Baker MB, et al., 2020, Bioprinting: From Tissue and Organ Development to In Vitro Models. Chem Rev, 120:10547. https://doi.org/10.1021/acs.chemrev.9b00789
7. Gutzweiler L, Kartmann S, Troendle K, et al., 2017, Large Scale Production and Controlled Deposition of Single HUVEC Spheroids for Bioprinting Applications. Biofabrication, 9:25027. https://doi.org/10.1088/1758-5090/aa7218
8. Tröndle K, Rizzo L, Pichler R, et al., 2021, Scalable Fabrication of Renal Spheroids and Nephron-like Tubules by Bioprinting and Controlled Self-assembly of Epithelial Cells. Biofabrication, 13:185. https://doi.org/10.1088/1758-5090/abe185
9. Ng WL, Chan A, Ong YS, et al., 2020, Deep Learning for Fabrication and Maturation of 3D Bioprinted Tissues and Organs. Virtual Phys Prototyp, 15:340. https://doi.org/10.1080/17452759.2020.1771741
10. Yu C, Jiang J, 2020, A Perspective on Using Machine Learning in 3D Bioprinting. Int J Bioprint, 6:253. https://doi.org/10.18063/ijb.v6i1.253
11. Benning L, Peintner A, Finkenzeller G, et al., 2020, Automated Spheroid Generation, Drug Application and Efficacy Screening Using a Deep Learning Classification: A Feasibility Study. Sci Rep, 10:11071. https://doi.org/10.1038/s41598-020-67960-0
12. La Greca A, Pérez N, Castañeda S, et al., 2020, Celldeath: A Tool for Detection of Cell Death in Transmitted Light Microscopy Images by Deep Learning-based Visual Recognition. PLoS One, 16:e0253666. https://doi.org/10.1101/2020.03.22.002253.
13. Kim SY, Moon A, 2012, Drug-induced Nephrotoxicity and its Biomarkers. Biomol Ther, 20:268. https://doi.org/10.4062/biomolther.2012.20.3.268
14. Yao X, Panichpisal K, Kurtzman N, et al., 2007, Cisplatin Nephrotoxicity: A Review. Am J Med Sci, 334:115. https://doi.org/10.1097/MAJ.0b013e31812dfe1e
15. Tröndle K, Koch F, Finkenzeller G, et al., 2019, Bioprinting of High Cell Density Constructs Leads to Controlled Lumen Formation with Self-assembly of Endothelial Cells. J Tissue Eng Regen Med, 13:1883–95. https://doi.org/10.1002/term.2939
16. Salas S, Mercier C, Ciccolini J, et al., 2006. Therapeutic Drug Monitoring for Dose Individualization of Cisplatin in Testicular Cancer Patients Based Upon Total Platinum Measurement in Plasma. Ther Drug Monit, 28:532–9. https://doi.org/10.1097/00007691-200608000-00008
17. King SM, Higgins JW, Nino CR, et al., 2017, 3D Proxima Tubule Tissues Recapitulate Key Aspects of Renal Physiology to Enable Nephrotoxicity Testing. Front Physiol, 8:123. https://doi.org/10.3389/fphys.2017.00123
18. Vormann MK, Gijzen L, Hutter S, et al., 2018, Nephrotoxicity and Kidney Transport Assessment on 3D Perfused Proximal Tubules. AAPS J, 20:90. https://doi.org/10.1208/s12248-018-0248-z
19. Han WK, Bailly V, Abichandani R, et al., 2002. Kidney Injury Molecule-1 (KIM-1): A Novel Biomarker for Human Renal Proximal Tubule Injury. Kidney Int, 62:237. https://doi.org/10.1046/j.1523-1755.2002.00433.x
20. Falkner S, Klein A, Hutter F. BOHB: Robust and Efficient Hyperparameter Optimization at Scale. 2018 International Conference on Machine Learning.
21. Boncler M, Różalski M, Krajewska U, et al., 2014, Comparison of PrestoBlue and MTT Assays of Cellular Viability in the Assessment of Anti-proliferative Effects of Plant Extracts on Human Endothelial Cells. J Pharmacol Toxicol Methods, 69:9. https://doi.org/10.1016/j.vascn.2013.09.003