Melt pool super solution reconstruction based on dual path deep learning for laser powder bed fusion monitoring
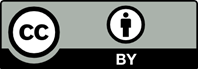
Melt pool monitoring in laser powder bed fusion (L-PBF) is an important foundation for process control and melting dynamics research. However, due to hardware limitations and the intense metallurgical phenomena during the melting process, the quality of the melt pool monitoring images cannot be guaranteed. This paper proposes a deep learning-based melt pool image super-resolution (SR) reconstruction method based on the particularity of melt pool images. It is an innovative dual-path structure. The first branch utilizes residual-in-residual structures and the efficient channel attention-Net attention mechanism to achieve the adaptive feature extraction of high-frequency information, thereby capturing effective melt pool boundary information. The second branch uses the U-Net structure to address the low utilization of overall characteristics of the melt pool in the chain-based SR network. In addition to the universal peak signal-to-noise ratio and structural similarity index measure metrics, the reconstruction accuracy of melt pool contour features is used to measure model performance, which intuitively reflects the significance of this work for melt pool monitoring. The results demonstrate that the proposed SR reconstruction method enhances the resolution and clarity of melt pool images. Furthermore, SR reconstruction of the melt pool effectively reduces errors in extracting melt pool features. This method provides a network paradigm for high-precision L-PBF monitoring. It integrates important boundary information and overall morphology features of the melt pool through a dual path structure, thereby achieving reliable SR reconstruction. This research will contribute to low-cost in situ monitoring of L-PBF and subsequent process control.
- Liu Y, Sing SL. A review of advances in additive manufacturing and the integration of high-performance polymers, alloys, and their composites. Mat Sci Addit Manuf. 2023;2(3):1587. doi: 10.36922/msam.1587
- Sehhat MH, Sutton AT, Hung CH, et al. Plasma spheroidization of gas-atomized 304L stainless steel powder for laser powder bed fusion process. Mat Sci Addit Manuf. 2022;1(1):1. doi: 10.18063/msam.v1i1.1
- Fé-Perdomo IL, Ramos-Grez JA, Beruvides G, Mujica RA. Selective laser melting: Lessons from medical devices industry and other applications. Rapid Prototyp J. 2021;27(10):1801-1830. doi: 10.1108/RPJ-07-2020-0151
- Lin X, Zhu K, Fuh JYH, Duan X. Metal-based additive manufacturing condition monitoring methods: From measurement to control. ISA Trans. 2022;120:147-166. doi: 10.1016/j.isatra.2021.03.001
- Wang Q, Lin X, Duan X, Yan R, Fuh JYH, Zhu K. Gaussian process classification of melt pool motion for laser powder bed fusion process monitoring. Mech Syst Signal Proc. 2023;198:110440. doi: 10.1016/j.ymssp.2023.110440
- Wang J, Zhu R, Liu Y, Zhang L. Understanding melt pool characteristics in laser powder bed fusion: An overview of single-and multi-track melt pools for process optimization. Adv Powder Mater. 2023;2(4):100137. doi: 10.1016/j.apmate.2023.100137
- Tao Z, Thanki A, Goossens L, Witvrouw A, Vrancken B, Dewulf W. Photodiode-based porosity prediction in laser powder bed fusion considering inter-hatch and inter-layer effects. J Mater Process Technol. 2024;332:118539. doi: 10.1016/j.jmatprotec.2024.118539
- Yang W, Qiu Y, Liu W, Qiu X, Bai Q. Defect prediction in laser powder bed fusion with the combination of simulated melt pool images and thermal images. J Manuf Process. 2023;106:214-222. doi: 10.1016/j.jmapro.2023.10.006
- Mao Y, Lin X, Zhu K. Selective laser melting monitoring based on the plume and its motion features. IEEE Trans Instrum Meas. 2024;73:1-14. doi: 10.1109/TIM.2024.3432145
- Jin M, Wu X, Shao W, et al. Preparation and microstructural evolution of spherical B-modified MoSi2 powders by induction plasma spheroidization. Ceram Int. 2022;48(14):20639-20647. doi: 10.1016/j.ceramint.2022.04.033
- Li Y, Chen Z, Lin L, Liu S, Wang H, Zhang J. Effect of pores on the stress field of high-frequency vibration of TC17 specimen manufactured by laser additive. Int J Fract. 2022;235(1):117-127. doi: 10.1007/s10704-021-00613-z
- Ince FD, Karna S, Zhang T, et al. An experimental process parameter study on the identification of defects in additively fabricated Al6061 with laser powder bed fusion. Mat Sci Addit Manuf. 2024;3(3):3652. doi: 10.36922/msam.3652
- Brion DAJ, Shen M, Pattinson SW. Automated recognition and correction of warp deformation in extrusion additive manufacturing. Addit Manuf. 2022;56:102838. doi: 10.1016/j.addma.2022.102838
- Xiao Y, Wang X, Yang W, et al. Data-driven prediction of future melt pool from built parts during metal additive manufacturing. Addit Manuf. 2024;93:104438. doi: 10.1016/j.addma.2024.104438
- McCann R, Obeidi MA, Hughes C, et al. In-situ sensing, process monitoring and machine control in laser powder bed fusion: A review. Addit Manuf. 2021;45:102058. doi: 10.1016/j.addma.2021.102058
- Taherkhani K, Ero O, Liravi F, Toorandaz S, Toyserkani E. On the application of in-situ monitoring systems and machine learning algorithms for developing quality assurance platforms in laser powder bed fusion: A review. J Manuf Process. 2023;99:848-897. doi: 10.1016/j.jmapro.2023.05.048
- Jiao W, Wang Q, Cheng Y, Zhang Y. End-to-end prediction of weld penetration: A deep learning and transfer learning based method. J Manuf Process. 2021;63:191-197. doi: 10.1016/j.jmapro.2020.01.044
- Chen Z, Chen J, Feng Z. Welding penetration prediction with passive vision system. J Manuf Process. 2018;36:224-230. doi: 10.1016/j.jmapro.2018.10.009
- Sampson R, Lancaster R, Sutcliffe M, Carswell D, Hauser C, Barras J. An improved methodology of melt pool monitoring of direct energy deposition processes. Opt Laser Technol. 2020;127:106194. doi: 10.1016/j.optlastec.2020.106194
- Barua S, Sparks T, Liou F. Development of low‐cost imaging system for laser metal deposition processes. Rapid Prototyp J. 2011;17(3):203-210. doi: 10.1108/13552541111124789
- Zhang S, Fu T, Jahn A, Collet A, Schleifenbaum JH. Towards deep-learning-based image enhancement for optical camera-based monitoring system of laser powder bed fusion process. Int J Comput Integr Manuf. 2023;36(9):1281-1294. doi: 10.1080/0951192X.2022.2104461
- Guo S, Liu Y, Cui L, et al. In-situ capture of melt pool signature in high-speed laser cladding using fully convolutional network. Opt Lasers Eng. 2024;176:108113. doi: 10.1016/j.optlaseng.2024.108113
- Fang Q, Tan Z, Li H, et al. In-situ capture of melt pool signature in selective laser melting using U-Net-based convolutional neural network. J Manuf Process. 2021;68:347-355. doi: 10.1016/j.jmapro.2021.05.052
- Al-Mekhlafi H, Liu S. Single image super-resolution: A comprehensive review and recent insight. Front Comput Sci. 2023;18(1):181702. doi: 10.1007/s11704-023-2588-9
- Zhou H, Yan L, Zhang L, Zheng R, Yu F. Improved SINGLE image Super-resolution Based on Edge Directed Interpolation. Vol. 9686. Eighth International Symposium on Advanced Optical Manufacturing and Testing Technology (AOMATT2016). SPIE; 2016.
- Huang D, Liu H. A short survey of image super resolution algorithms. J Comput Sci Technol Updates. 2015;2(2):19-29.
- Chauhan K, Patel SN, Kumhar M, et al. Deep learning-based single-image super-resolution: A comprehensive review. IEEE Access. 2023;11:21811-21830. doi: 10.1109/ACCESS.2023.3251396
- Dong C, Loy CC, He K, Tang X. Learning a deep convolutional network for image super-resolution. In: Computer Vision- ECCV 2014. Germany: Springer; 2014. p. 184-199. doi: 10.1007/978-3-319-10593-2_13
- Dong C, Loy CC, Tang X. Accelerating the super-resolution convolutional neural network. In: Computer Vision-ECCV 2016. Germany: Springer; 2016. p. 391-407. doi: 10.1007/978-3-319-46475-6_25
- Kim J, Lee JK, Lee KM. Accurate Image Super-Resolution Using Very Deep Convolutional Networks. 2016 IEEE Conference on Computer Vision and Pattern Recognition (CVPR); 2016. p. 1646-1654. doi: 10.1109/CVPR.2016.182
- Lim B, Son S, Kim H, Nah S, Lee KM. Enhanced Deep Residual Networks for Single Image Super-Resolution. In: 2017 IEEE Conference on Computer Vision and Pattern Recognition Workshops (CVPRW); 2017. p. 1132-1140. doi: 10.1109/CVPRW.2017.151
- Zhang Y, Li K, Li K, Wang L, Zhong B, Fu Y. Image Super-resolution using very deep residual channel attention networks. Computer Vision-ECCV 2018. Germany: Springer; 2018. 294-310. doi: 10.1007/978-3-030-01234-2_18
- Guo Y, Chen J, Wang J, et al. Closed-Loop Matters: Dual Regression Networks for Single Image Super-Resolution. 2020 IEEE/CVF Conference on Computer Vision and Pattern Recognition (CVPR); 2020. p. 5406-5415. doi: 10.1109/CVPR42600.2020.00545
- Du G, Zhang P, Guo J, et al. DERE-Net: A dual-encoder residual enhanced U-Net for muscle fiber segmentation of H and E images. Biomed Signal Process Control. 2024;98:106765. doi: 10.1016/j.bspc.2024.106765
- Ledig C, Theis L, Huszár F, et al. Photo-Realistic Single Image Super-resolution Using a Generative Adversarial Network. In: 2017 IEEE Conference on Computer Vision and Pattern Recognition (CVPR); 2017. p. 105-114. doi: 10.1109/CVPR.2017.19
- Sun S, Peng X, Cao H. Accurate inspection and super-resolution reconstruction for additive manufactured defects based on stokes vector method and deep learning. Photonics. 2024;11(9):874. doi: 10.3390/photonics11090874
- Zhu W, Li H, Shen S, et al. In-situ monitoring additive manufacturing process with AI edge computing. Opt Laser Technol. 2024;171:110423. doi: 10.1016/j.optlastec.2023.110423
- Timofte R, Agustsson E, Gool LV, et al. NTIRE 2017 Challenge on Single Image Super-resolution: Methods and Results. In: 2017 IEEE Conference on Computer Vision and Pattern Recognition Workshops (CVPRW). 2017. p. 1110-1121. doi: 10.1109/CVPRW.2017.149
- Wang Q, Wu B, Zhu P, Li P, Zuo W, Hu Q. ECA-Net: Efficient channel attention for deep convolutional neural networks. In: 2020 IEEE/CVF Conference on Computer Vision and Pattern Recognition (CVPR); 2020. p. 11531-11539. doi: 10.1109/CVPR42600.2020.01155
- Zhu Y, Geiß C, So E. Image super-resolution with dense-sampling residual channel-spatial attention networks for multi-temporal remote sensing image classification. Int J Appl Earth Obs Geoinf. 2021;104:102543. doi: 10.1016/j.jag.2021.102543
- Woo S, Park J, Lee JY, Kweon IS. CBAM: Convolutional Block Attention Module. Computer Vision-ECCV 2018: 15th European Conference, Munich, Germany, September 8-14, 2018, Proceedings, Part VII; 2018. p. 3-19. doi: 10.1007/978-3-030-01234-2_1
- Hu J, Shen L, Sun G. Squeeze-and-Excitation Networks. 2018 IEEE/CVF Conference on Computer Vision and Pattern Recognition; 2018. p. 7132-7141. doi: 10.1109/CVPR.2018.00745
- He Y, Cai C, Chen G, Hu J, Hu S, Fu J. PFEI-Net: A profound feature exploration and interaction network for ceramic substrate surface defect detection. Expert Syst Appl. 2025;263:125741. doi: 10.1016/j.eswa.2024.125741
- Shi W, Caballero J, Huszár F, et al. Real-Time Single Image and Video Super-Resolution Using an Efficient Sub-Pixel Convolutional Neural Network. 2016 IEEE Conference on Computer Vision and Pattern Recognition (CVPR); 2016. p. 1874-1883. doi: 10.1109/CVPR.2016.207
- Liu Q, Zhao J. MA-Res U-Net: Design of soybean navigation system with improved U-Net Model. Phyton Int J Exp Bot. 2024;93(10):2663-2681. doi: 10.32604/phyton.2024.056054