Analyzing Cell-Scaffold Interaction through Unsupervised 3D Nuclei Segmentation
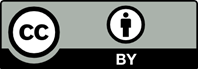
Fibrous scaffolds have been extensively used in three-dimensional (3D) cell culture systems to establish in vitro models in cell biology, tissue engineering, and drug screening. It is a common practice to characterize cell behaviors on such scaffolds using confocal laser scanning microscopy (CLSM). As a noninvasive technology, CLSM images can be utilized to describe cell-scaffold interaction under varied morphological features, biomaterial composition, and internal structure. Unfortunately, such information has not been fully translated and delivered to researchers due to the lack of effective cell segmentation methods. We developed herein an end-to-end model called Aligned Disentangled Generative Adversarial Network (AD-GAN) for 3D unsupervised nuclei segmentation of CLSM images. AD-GAN utilizes representation disentanglement to separate content representation (the underlying nuclei spatial structure) from style representation (the rendering of the structure) and align the disentangled content in the latent space. The CLSM images collected from fibrous scaffold-based culturing A549, 3T3, and HeLa cells were utilized for nuclei segmentation study. Compared with existing commercial methods such as Squassh and CellProfiler, our AD-GAN can effectively and efficiently distinguish nuclei with the preserved shape and location information. Building on such information, we can rapidly screen cell-scaffold interaction in terms of adhesion, migration and proliferation, so as to improve scaffold design.
1. Ravi M, Paramesh V, Kaviya SR., et al. 2015. 3D Cell Culture Systems: Advantages and Applications. J Cell Physiol, 230:16–26. https://doi.org/10.1002/jcp.24683
2. Pampaloni F, Reynaud EG, Stelzer EH, 2007. The Third Dimension Bridges the Gap between Cell Culture and Live Tissue. Nat Rev Mol Cell Biol, 8:839–45. https://doi.org/10.1038/nrm2236
3. Paddock SW, 1999. Confocal Laser Scanning Microscopy. Biotechniques, 27:992–1004. https://doi.org/10.2144/99275ov01
4. Al-Kofahi Y, Zaltsman A, Graves R, et al. 2018. A Deep Learning-based Algorithm for 2-D Cell Segmentation in Microscopy Images. BMC Bioinformatics, 19:1–11.
5. Vahadane A and Sethi A, 2013. Towards Generalized Nuclear Segmentation in Histological Images. In: 13th IEEE International Conference on Bioinformatics and Bioengineering. p1-4.
6. Xue JH, Titterington DM, 2011. t-Tests, f-Tests and Otsu’s methods for image thresholding. IEEE Trans Image Proc, 20:2392–96. https://doi.org/10.1109/TIP.2011.2114358
7. Jie S, Hong GS, Rahman M, et al., 2002. Feature Extraction and Selection in tool Condition Monitoring System. In: Australian Joint Conference on Artificial Intelligence. Berlin, Heidelberg: Springer. p487-497.
8. Veta M, Van Diest PJ, Kornegoor R, et al. 2013. Automatic Nuclei Segmentation in H&E Stained Breast Cancer Histopathology Images. PLoS One, 8:e70221. https://doi.org/10.1371/journal.pone.0070221
9. Zhang C, Sun C, Pham TD, 2013. Segmentation of Clustered Nuclei Based on Concave Curve Expansion. J Microsc, 251:57–67. https://doi.org/10.1111/jmi.12043
10. Xing F, Yang L, 2016. Robust Nucleus/Cell Detection and Segmentation in Digital Pathology and Microscopy Images: A Comprehensive Review. IEEE Rev Biomed Eng, 9:234–63. https://doi.org/10.1109/RBME.2016.2515127
11. Yang Z, Bogovic JA, Carass A, et al. 2013. Automatic Cell Segmentation in Fluorescence Images of Confluent Cell Monolayers Using Multi-object Geometric Deformable Model. Proc SPIE Int Soc Opt Eng, 8669:2006603. https://doi.org/10.1117/12.2006603
12. McQuin C, Goodman A, Chernyshev V, et al. 2018. CellProfiler 3.0: Next-generation Image Processing for Biology. PLoS Biol, 16:e2005970. https://doi.org/10.1371/journal.pbio.2005970
13. Rizk A, Paul G, Incardona P, et al., 2014. Segmentation and Quantification of Subcellular Structures in Fluorescence Microscopy Images Using Squassh. Nat Protoc, 9:6–596. https://doi.org/10.1038/nprot.2014.037
14. Kumar N, Verma R, Sharma S, et al., 2017. A Dataset and a Technique for Generalized Nuclear Segmentation for Computational Pathology. IEEE Trans Med Imaging, 36:1550–60.
15. Xing F, Xie Y, Yang L, 2015. An Automatic Learning-based Framework for Robust Nucleus Segmentation. IEEE Trans Med Imaging, 35:550–66.
16. Dunn KW, Fu C, Ho DJ, et al. 2019. DeepSynth: Three dimensional Nuclear Segmentation of Biological Images Using Neural Networks Trained with Synthetic Data. Sci Rep, 9:1–15.
17. Liu D, Zhang D, Song Y, et al. 2020. Unsupervised Instance Segmentation in Microscopy Images Via Panoptic Domain Adaptation and Task Re-weighting. In: Proceedings of the IEEE/CVF Conference on Computer Vision and Pattern Recognition. p4243-4252.
18. Hou L, Agarwal A, Samaras D, et al., 2019. Robust Histopathology Image Analysis: To Label or to Synthesize? In: Proceedings of the IEEE/CVF Conference on Computer Vision and Pattern Recognition. p8533-8542.
19. Yang J, Dvornek NC, Zhang F, et al., 2019. Unsupervised Domain Adaptation Via Disentangled Representations: Application to Cross-modality Liver Segmentation. In: International Conference on Medical Image Computing and Computer-Assisted Intervention. p255-263.
20. Sun J, Jing L, Liu H, et al., 2020. Generating Nanotopography on PCL Microfiber Surface for Better Cell-Scaffold Interactions. Proc. Manufact., 48:619–24.
21. Jing L, Wang X, Leng B, et al., 2021. Engineered Nanotopography on the Microfibers of 3D-Printed PCL Scaffolds to Modulate Cellular Responses and Establish an In Vitro Tumor Model. ACS Appl Biomater, 4:1381–94.
22. Kingma DP, Ba J, 2014. Adam: A Method for Stochastic Optimization. arXiv, 2014:6980. https://arxiv.org/abs/1412.6980
23. Zhu JY, Park T, Isola P, et al., 2017. Unpaired Image-to-image Translation Using Cycle-consistent Adversarial Networks. In: Proceedings of the IEEE International Conference on Computer Vision. p2223–32.
24. Mao X, Li Q, Xie H, et al., 2017. Least Squares Generative Adversarial Networks. In: Proceedings of the IEEE International Conference on Computer Vision. p2794–802.
25. Boutin ME, Voss TC, Titus SA, et al., 2018. A High throughput Imaging and Nuclear Segmentation Analysis Protocol for Cleared 3D Culture Models. Sci Rep, 8:1–14.
26. Milletari F, Navab N, Ahmadi SA, 2016. V-net: Fully Convolutional Neural Networks for Volumetric Medical Image Segmentation. In: 2016 4th International Conference on 3D Vision. p565–71.
27. Ker J, Wang L, Rao J, et al., 2017. Deep Learning Applications in Medical Image Analysis. IEEE Access, 6:9375–89.
28. Kromp F, Bozsaky E, Rifatbegovic F, et al., 2020. An Annotated Fluorescence Image Dataset for Training Nuclear Segmentation Methods. Sci Data, 7:1–8.
29. Sun J, Vijayavenkataraman S, Liu H, 2017. An Overview of Scaffold Design and Fabrication Technology for Engineered Knee Meniscus. Materials, 10:29. https://doi.org/10.3390/ma10010029
30. Murphy MB, Blashki D, Buchanan RM, et al., 2012. Adult and Umbilical Cord Blood-derived Platelet-rich Plasma for Mesenchymal Stem Cell Proliferation, Chemotaxis, and Cryo-preservation. Biomaterials, 33:5308–16. https://doi.org/10.1016/j.biomaterials.2012.04.007
31. Salem AK, Stevens R, Pearson RG, et al., 2002. Interactions of 3T3 Fibroblasts and Endothelial Cells with Defined Pore Features. J Biomed Mater Res, 61:212–7. https://doi.org/10.1002/jbm.10195
32. Jing L, Sun J, Liu H, et al., 2021. Using Plant Proteins to Develop Composite Scaffolds for Cell Culture Applications. Int J Bioprint, 7:298. https://doi.org/10.18063/ijb.v7i1.298
33. Jiang RD, Shen H, Piao YJ, 2010. The Morphometrical Analysis on the Ultrastructure of A549 Cells. Rom J Morphol Embryol, 51:663–7.
34. Theiszova M, Jantova S, Dragunova J, et al., 2005. Comparison the Cytotoxicity of Hydroxiapatite Measured by Direct Cell Counting and MTT Test in Murine Fibroblast NIH-3T3 Cells. Biomed Papers Palacky Univ Olomouc, 149:393.
35. Pawley J, 2006. Handbook of Biological Confocal Microscopy. Vol. 236. Berlin: Springer Science and Business Media.
36. Tasnadi EA, Toth T, Kovacs M, et al., 2020. 3D-Cell-Annotator: An Open-source Active Surface Tool for Single-cell Segmentation in 3D Microscopy Images. Bioinformatics, 36:2948–9. https://doi.org/10.1093/bioinformatics/btaa029