On the in silico application of the center-of-mass distance method
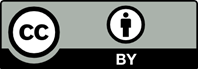
This study aims to protocolize the utilization of the center-of-mass (CoM) distance method in GROMACS MD simulation software as a useful method for evaluating the binding affinity change in heterodimeric protein due to induced changes in one of the units. The hypothesis underlines the basic principles in biophysics, that an increase of the binding affinity is expected to reduce the relative CoM distance between monomers, while the opposite is expected to increase the relative CoM distance. However, it has been found that the CoM distance analysis must be strictly preformed during the convergent phase of systems’ dynamics, once the monomers enter mutually stable conformation — a limitation which has usually been overlooked. The method was used to study the impact of K417Y severe acute respiratory syndrome coronavirus 2 (SARS-CoV-2) surface glycoprotein (S-protein) mutation. It has been found that the K417Y mutation favors reduced binding affinity between SARS-CoV-2 S-protein and human angiotensin-converting enzyme 2 (hACE2) receptor, which is due to the loss of the permanent K417-D30 salt bridge in favor of a temporary Y417-D30 hydrogen bond. The destabilizing impact of K417Y mutation on S-protein–hACE2 complex was confirmed by radius of gyration analysis.
- Stojanov D. Structural implications of SARS-CoV-2 surface glycoprotein N501Y mutation within receptor-binding domain [499-505]-computational analysis of the most frequent Asn501 polar uncharged amino acid mutations. Biotechnol Biotechnol Equip. 2023;37(1):2206492. doi: 10.1080/13102818.2023.2206492
- Ibrahim MA, Abdelrahman AH, Mohamed TA, et al. In silico mining of terpenes from red-sea invertebrates for SARS-CoV-2 main protease (Mpro) inhibitors. Molecules. 2021;26(7):2082. doi: 10.3390/molecules26072082
- Kumar R, Murugan NA, Srivastava V. Improved binding affinity of omicron’s spike protein for the human angiotensin-converting enzyme 2 receptor is the key behind its increased virulence. Int J Mol Sci. 2022;23(6):3409. doi: 10.3390/ijms23063409
- Carter C, Airas J, Parish CA. Atomistic insights into the binding of SARS-CoV-2 spike receptor binding domain with the human ACE2 receptor: The importance of residue 493. J Mol Graph Model. 2023;118:108360. doi: 10.1016/j.jmgm.2022.108360
- Tian F, Tong B, Sun L, et al. N501Y mutation of spike protein in SARS-CoV-2 strengthens its binding to receptor ACE2. Elife. 2021;10:e69091. doi: 10.7554/eLife.69091
- Godschalk F, Genheden S, Söderhjelm P, Ryde U. Comparison of MM/GBSA calculations based on explicit and implicit solvent simulations. Phys Chem Chem Phys. 2013;15(20):7731-7739. doi: 10.1039/C3CP00116D
- Stojanov D. Phylogenicity of B.1.1.7 surface glycoprotein, novel distance function and first report of V90T missense mutation in SARS-CoV-2 surface glycoprotein. Meta Gene. 2021;30:100967. doi: 10.1016/j.mgene.2021.100967
- Stojanov D. Data on multiple SARS-CoV-2 surface glycoprotein alignments. Data Brief. 2021;38:107414. doi: 10.1016/j.dib.2021.107414
- Deng JH, Luo J, Mao YL, et al. π-π stacking interactions: Non-negligible forces for stabilizing porous supramolecular frameworks. Sci Adv. 2020;6(2):eaax9976. doi: 10.1126/sciadv.aax9976
- Ahamad S, Ali H, Secco I, Giacca M, Gupta D. Anti-fungal drug anidulafungin inhibits SARS-CoV-2 spike-induced syncytia formation by targeting ACE2-spike protein interaction. Front Genet. 2022;13:866474. doi:10.3389/fgene.2022.866474
- Ahamad S, Gupta D, Kumar V. Targeting SARS-CoV-2 nucleocapsid oligomerization: Insights from molecular docking and molecular dynamics simulations. J Biomol Struct Dyn. 2022;40(6):2430-2443. doi: 10.1080/07391102.2020.1839563
- Ahamad S, Hema K, Ahmad S, Kumar V, Gupta D. Insights into the structure and dynamics of SARS-CoV-2 spike glycoprotein double mutant L452R-E484Q. 3 Biotech. 2022;12(4):87. doi: 10.1007/s13205-022-03151-0
- Ahamad S, Hema K, Gupta D. Structural stability predictions and molecular dynamics simulations of RBD and HR1 mutations associated with SARS-CoV-2 spike glycoprotein. J Biomol Struct Dyn. 2022;40(15):6697-6709. doi: 10.1080/07391102.2021.1889671
- Ahamad S, Kanipakam H, Gupta D. Insights into the structural and dynamical changes of spike glycoprotein mutations associated with SARS-CoV-2 host receptor binding. J Biomol Struct Dyn. 2022;40(1):263-275. doi: 10.1080/07391102.2020.1811774
- Lan J, Ge J, Yu J, et al. Structure of the SARS-CoV-2 spike receptor-binding domain bound to the ACE2 receptor. Nature. 2020;581(7807):215-220. doi: 10.1038/s41586-020-2180-5
- Brooks BR, Bruccoleri RE, Olafson BD, States DJ, Volume X Issue X (2024) 8 https://doi.org/10.36922/gpd.2657 Swaminathan SA, Karplus M. CHARMM: A program for macromolecular energy, minimization, and dynamics calculations. J Comput Chem. 1983;4(2):187-217. doi: 10.1002/jcc.540040211
- Curry HB. The method of steepest descent for non-linear minimization problems. Q Appl Math. 1944;2(3):258-261. doi: 10.1090/qam/10667
- Abraham MJ, Murtola T, Schulz R, et al. GROMACS: High performance molecular simulations through multi-level parallelism from laptops to supercomputers. SoftwareX. 2015;1-2:19-25. doi: 10.1016/j.softx.2015.06.001
- Clementel D, Del Conte A, Monzon AM, et al. RING 3.0: Fast generation of probabilistic residue interaction networks from structural ensembles. Nucleic Acids Res. 2022;50(W1):W651-W656. doi: 10.1093/nar/gkac365
- Kumar S, Nussinov R. Close‐range electrostatic interactions in proteins. ChemBioChem. 2002;3(7):604-617. doi: 10.1002/1439-7633(20020703)3:7<604::AID-CBIC604>3.0.CO;2-X
- Baker EN, Hubbard RE. Hydrogen bonding in globular proteins. Prog Biophys Mol Biol. 1984;44(2):97-179. doi: 10.1016/0079-6107(84)90007-5