Current status and future potential of radiomics in the management of patients with gastric cancer
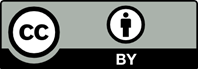
Gastric cancer (GC) is one of the most common malignant tumors in the world and has attracted much attention due to its high incidence and mortality. Early detection and accurate diagnosis of GC is very important to improve the survival rate of patients. Traditional imaging techniques, such as computed tomography (CT), have played an important role in the diagnosis and staging of GC, but their accuracy and predictive power are still limited. Radiomics, as an emerging multidisciplinary field, combines medical imaging, computer technology, and bioinformatics to reveal potential biological information in image data through quantitative extraction of image features. To review the current status and future potential of radiomics in the diagnosis, prognosis, and outcome prediction for patients with GC, related studies with keywords such as “radiomics,” “gastric cancer,” and “predict” were retrieved from PubMed (until October 2022). The clinical value of radiomics in GC research, which encompasses diagnosis, clinical staging, and prognostic prediction, was analyzed and summarized. A total of 52 original articles in radiomics were selected for the prediction of diagnostic classification (n = 9), tumor-node-metastasis stages (n = 19), prognosis and response prediction to treatment (n = 20), and the use of deep learning algorithm (n = 4). Among these, 39 (75%) were single-center studies and 13 (25%) were multicenter studies, with sample sizes ranging from 47 to 1,778. In terms of imaging modalities, 44 studies utilized CT-based radiomics, six used positron emission tomography-CT, and a few employed magnetic resonance imaging. With the continuous improvement and development in radiomics, it has great potential in the diagnosis, post-treatment follow-up, and treatment decision for patients with GC.
- Bray F, Ferlay J, Soerjomataram I, Siegel RL, Torre LA, Jemal A. Global cancer statistics 2018: GLOBOCAN estimates of incidence and mortality worldwide for 36 cancers in 185 countries. CA Cancer J Clin. 2018;68(6):394-424. doi: 10.3322/caac.21492
- Sung H, Ferlay J, Siegel RL, et al. Global cancer statistics 2020: GLOBOCAN estimates of incidence and mortality worldwide for 36 cancers in 185 countries. CA Cancer J Clin. 2021;71(3):209-249. doi: 10.3322/caac.21660
- Noh SH, Park SR, Yang HK, et al. Adjuvant capecitabine plus oxaliplatin for gastric cancer after D2 gastrectomy (CLASSIC): 5-year follow-up of an open-label, randomised phase 3 trial. Lancet Oncol. 2014;15(12):1389-1396. doi: 10.1016/S1470-2045(14)70473-5
- Spolverato G, Ejaz A, Kim Y, et al. Rates and patterns of recurrence after curative intent resection for gastric cancer: A United States multi-institutional analysis. J Am Coll Surg. 2014;219(4):664-675. doi: 10.1016/j.jamcollsurg.2014.03.062
- Deng J, Liang H, Wang D, Sun D, Pan Y, Liu Y. Investigation of the recurrence patterns of gastric cancer following a curative resection. Surg Today. 2011;41(2):210-215. doi: 10.1007/s00595-009-4251-y
- Takahashi T, Saikawa Y, Kitagawa Y. Gastric cancer: Current status of diagnosis and treatment. Cancers (Basel). 2013;5(1):48-63. doi: 10.3390/cancers5010048
- Pasechnikov V, Chukov S, Fedorov E, Kikuste I, Leja M. Gastric cancer: Prevention, screening and early diagnosis. World J Gastroenterol. 2014;20(38):13842-13862. doi: 10.3748/wjg.v20.i38.13842
- Matsuoka T, Yashiro M. Biomarkers of gastric cancer: Current topics and future perspective. World J Gastroenterol. 2018;24(26):2818-2832. doi: 10.3748/wjg.v24.i26.2818
- Machlowska J, Baj J, Sitarz M, Maciejewski R, Sitarz R. Gastric cancer: Epidemiology, risk factors, classification, genomic characteristics and treatment strategies. Int J Mol Sci. 2020;21(11):4012. doi: 10.3390/ijms21114012
- Seevaratnam R, Cardoso R, McGregor C, et al. How useful is preoperative imaging for tumor, node, metastasis (TNM) staging of gastric cancer? A meta-analysis. Gastric Cancer. 2012;15(Suppl 1):S3-S18. doi: 10.1007/s10120-011-0069-6
- Johnston FM, Beckman M. Updates on management of gastric cancer. Curr Oncol Rep. 2019;21(8):67. doi: 10.1007/s11912-019-0820-4
- Bi WL, Hosny A, Schabath MB, et al. Artificial intelligence in cancer imaging: Clinical challenges and applications. CA Cancer J Clin. 2019;69(2):127-157. doi: 10.3322/caac.21552
- Gillies RJ, Kinahan PE, Hricak H. Radiomics: Images are more than pictures, they are data. Radiology. 2016;278(2):563-577. doi: 10.1148/radiol.2015151169
- Aerts HJ, Velazquez ER, Leijenaar RT, et al. Decoding tumour phenotype by noninvasive imaging using a quantitative radiomics approach. Nat Commun. 2014;5:4006. doi: 10.1038/ncomms5006
- Wang Y, Jin ZY. Radiomics approaches in gastric cancer: A frontier in clinical decision making. Chin Med J (Engl). 2019;132(16):1983-1989. doi: 10.1097/CM9.0000000000000360
- Zhang W, Fang M, Dong D, et al. Development and validation of a CT-based radiomic nomogram for preoperative prediction of early recurrence in advanced gastric cancer. Radiother Oncol. 2020;145:13-20. doi: 10.1016/j.radonc.2019.11.023
- Jiang Y, Chen C, Xie J, et al. Radiomics signature of computed tomography imaging for prediction of survival and chemotherapeutic benefits in gastric cancer. EBioMedicine. 2018;36:171-182. doi: 10.1016/j.ebiom.2018.09.007
- Smyth EC, Nilsson M, Grabsch HI, van Grieken NC, Lordick F. Gastric cancer. Lancet. 2020;396(10251):635-648. doi: 10.1016/S0140-6736(20)31288-5
- Zhang Q, Peng C. Cancer-associated fibroblasts regulate the biological behaviour of cancer cells and stroma in gastric cancer. Oncol Lett. 2018;15(1):691-698. doi: 10.3892/ol.2017.7385
- Ma Z, Fang M, Huang Y, et al. CT-based radiomics signature for differentiating Borrmann type IV gastric cancer from primary gastric lymphoma. Eur J Radiol. 2017;91:142-147. doi: 10.1016/j.ejrad.2017.04.007
- Sun YW, Ji CF, Wang H, et al. Differentiating gastric cancer and gastric lymphoma using texture analysis (TA) of positron emission tomography (PET). Chin Med J (Engl). 2020;134(4):439-447. doi: 10.1097/CM9.0000000000001206
- Sun ZQ, Hu SD, Li J, Wang T, Duan SF, Wang J. Radiomics study for differentiating gastric cancer from gastric stromal tumor based on contrast-enhanced CT images. J Xray Sci Technol. 2019;27(6):1021-1031. doi: 10.3233/XST-190574
- Wang R, Liu H, Liang P, Zhao H, Li L, Gao J. Radiomics analysis of CT imaging for differentiating gastric neuroendocrine carcinomas from gastric adenocarcinomas. Eur J Radiol. 2021;138:109662. doi: 10.1016/j.ejrad.2021.109662
- Kim BS, Oh ST, Yook JH, Kim BS. Signet ring cell type and other histologic types: Differing clinical course and prognosis in T1 gastric cancer. Surgery. 2014;155(6):1030-1035. doi: 10.1016/j.surg.2013.08.016
- Van der Post RS, Gullo I, Oliveira C, et al. Histopathological, molecular, and genetic profile of hereditary diffuse gastric cancer: Current knowledge and challenges for the future. Adv Exp Med Biol. 2016;908:371-391. doi: 10.1007/978-3-319-41388-4_18
- Zhang Y, Chen J, Liu S, et al. Assessment of histological differentiation in gastric cancers using whole-volume histogram analysis of apparent diffusion coefficient maps. J Magn Reson Imaging. 2017;45(2):440-449. doi: 10.1002/jmri.25360
- Li Q, Qi L, Feng QX, et al. Machine learning-based computational models derived from large-scale radiographic-radiomic images can help predict adverse histopathological status of gastric cancer. Clin Transl Gastroenterol. 2019;10(10):e00079. doi: 10.14309/ctg.0000000000000079
- Smyth EC, Verheij M, Allum W, et al. Gastric cancer: ESMO clinical practice guidelines for diagnosis, treatment and follow-up. Ann Oncol. 2016;27(Suppl 5):v38-v49. doi: 10.1093/annonc/mdw350
- Chen YC, Fang WL, Wang RF, et al. Clinicopathological variation of lauren classification in gastric cancer. Pathol Oncol Res. 2016;22(1):197-202. doi: 10.1007/s12253-015-9996-6
- Wang XX, Ding Y, Wang SW, et al. Intratumoral and peritumoral radiomics analysis for preoperative Lauren classification in gastric cancer. Cancer Imaging. 2020;20(1):83. doi: 10.1186/s40644-020-00358-3
- Wang Y, Liu W, Yu Y, et al. Potential value of CT radiomics in the distinction of intestinal-type gastric adenocarcinomas. Eur Radiol. 2020;30(5):2934-2944. doi: 10.1007/s00330-019-06629-3
- Chen T, Wu J, Cui C, et al. CT-based radiomics nomograms for preoperative prediction of diffuse-type and signet ring cell gastric cancer: A multicenter development and validation cohort. J Transl Med. 2022;20(1):38. doi: 10.1186/s12967-022-03232-x
- Edge SB, Byrd DR, Compton CC, et al. AJCC Cancer Staging Manual. 7th ed. Berlin: Springer; 2009.
- Kumagai K, Sano T. Revised points and disputed matters in the eighth edition of the TNM staging system for gastric cancer. Jpn J Clin Oncol. 2021;51(7):1024-1027. doi: 10.1093/jjco/hyab069
- Chen W, Wang S, Dong D, et al. Evaluation of lymph node metastasis in advanced gastric cancer using magnetic resonance imaging-based radiomics. Front Oncol. 2019;9:1265. doi: 10.3389/fonc.2019.01265
- Jiang Y, Wang W, Chen C, et al. radiomics signature on computed tomography imaging: Association with lymph node metastasis in patients with gastric cancer. Front Oncol. 2019;9:340. doi: 10.3389/fonc.2019.00340
- Feng QX, Liu C, Qi L, et al. An intelligent clinical decision support system for preoperative prediction of lymph node metastasis in gastric cancer. J Am Coll Radiol. 2019;16(7):952-960. doi: 10.1016/j.jacr.2018.12.017
- Wang Y, Liu W, Yu Y, et al. Prediction of the depth of tumor invasion in gastric cancer: Potential role of CT radiomics. Acad Radiol. 2020;27(8):1077-1084. doi: 10.1016/j.acra.2019.10.020
- Gao X, Ma T, Cui J, et al. A CT-based radiomics model for prediction of lymph node metastasis in early stage gastric cancer. Acad Radiol. 2021;28(6):e155-e164. doi: 10.1016/j.acra.2020.03.045
- Gao X, Ma T, Cui J, et al. A radiomics-based model for prediction of lymph node metastasis in gastric cancer. Eur J Radiol. 2020;129:109069. doi: 10.1016/j.ejrad.2020.109069
- Wang Y, Liu W, Yu Y, et al. CT radiomics nomogram for the preoperative prediction of lymph node metastasis in gastric cancer. Eur Radiol. 2020;30(2):976-986. doi: 10.1007/s00330-019-06398-z
- Yardimci AH, Sel I, Bektas CT, et al. Computed tomography texture analysis in patients with gastric cancer: A quantitative imaging biomarker for preoperative evaluation before neoadjuvant chemotherapy treatment. Jpn J Radiol. 2020;38(6):553-560. doi: 10.1007/s11604-020-00936-2
- Yang J, Wu Q, Xu L, et al. Integrating tumor and nodal radiomics to predict lymph node metastasis in gastric cancer. Radiother Oncol. 2020;150:89-96. doi: 10.1016/j.radonc.2020.06.004
- Wang L, Gong J, Huang X, et al. CT-based radiomics nomogram for preoperative prediction of No.10 lymph nodes metastasis in advanced proximal gastric cancer. Eur J Surg Oncol. 2021;47(6):1458-1465. doi: 10.1016/j.ejso.2020.11.132
- Sun Z, Jiang Y, Chen C, et al. Radiomics signature based on computed tomography images for the preoperative prediction of lymph node metastasis at individual stations in gastric cancer: A multicenter study. Radiother Oncol. 2021;165:179-190. doi: 10.1016/j.radonc.2021.11.003
- Wang X, Li C, Fang M, et al. Integrating No.3 lymph nodes and primary tumor radiomics to predict lymph node metastasis in T1-2 gastric cancer. BMC Med Imaging. 2021;21(1):58. doi: 10.1186/s12880-021-00587-3
- Liu Q, Li J, Xin B, et al. 18F-FDG PET/CT radiomics for preoperative prediction of lymph node metastases and nodal staging in gastric cancer. Front Oncol. 2021;11:723345. doi: 10.3389/fonc.2021.723345
- Xue XQ, Yu WJ, Shao XL, et al. Radiomics model based on preoperative 18F-fluorodeoxyglucose PET predicts N2-3b lymph node metastasis in gastric cancer patients. Nucl Med Commun. 2022;43(3):340-349. doi: 10.1097/MNM.0000000000001523
- Montagnani F, Crivelli F, Aprile G, et al. Long-term survival after liver metastasectomy in gastric cancer: Systematic review and meta-analysis of prognostic factors. Cancer Treat Rev. 2018;69:11-20. doi: 10.1016/j.ctrv.2018.05.010
- Chen X, Yang Z, Yang J, et al. Radiomics analysis of contrast-enhanced CT predicts lymphovascular invasion and disease outcome in gastric cancer: A preliminary study. Cancer Imaging. 2020;20(1):24. doi: 10.1186/s40644-020-00302-5
- Yardımcı AH, Koçak B, Turan Bektaş C, et al. Tubular gastric adenocarcinoma: Machine learning-based CT texture analysis for predicting lymphovascular and perineural invasion. Diagn Interv Radiol. 2020;26(6):515-522. doi: 10.5152/dir.2020.19507
- Zheng H, Zheng Q, Jiang M, et al. Contrast-enhanced CT based radiomics in the preoperative prediction of perineural invasion for patients with gastric cancer. Eur J Radiol. 2022;154:110393. doi: 10.1016/j.ejrad.2022.110393
- Fan L, Li J, Zhang H, et al. Machine learning analysis for the noninvasive prediction of lymphovascular invasion in gastric cancer using PET/CT and enhanced CT-based radiomics and clinical variables. Abdom Radiol (NY). 2022;47(4):1209-1222. doi: 10.1007/s00261-021-03315-1
- Yang L, Chu W, Li M, et al. Radiomics in gastric cancer: First clinical investigation to predict lymph vascular invasion and survival outcome using 18F-FDG PET/CT images. Front Oncol. 2022;12:836098. doi: 10.3389/fonc.2022.836098
- Bando E, Makuuchi R, Tokunaga M, Tanizawa Y, Kawamura T, Terashima M. Impact of clinical tumor-node-metastasis staging on survival in gastric carcinoma patients receiving surgery. Gastric Cancer. 2017;20(3):448-456. doi: 10.1007/s10120-016-0637-x
- Sohn BH, Hwang JE, Jang HJ, et al. Clinical significance of four molecular subtypes of gastric cancer identified by the cancer genome atlas project. Clin Cancer Res. 2017;23(15):4441-4449. doi: 10.1158/1078-0432.CCR-16-2211
- Bortolotto C, Lancia A, Stelitano C, et al. Radiomics features as predictive and prognostic biomarkers in NSCLC. Expert Rev Anticancer Ther. 2021;21(3):257-266. doi: 10.1080/14737140.2021.1852935
- Giganti F, Antunes S, Salerno A, et al. Gastric cancer: Texture analysis from multidetector computed tomography as a potential preoperative prognostic biomarker. Eur Radiol. 2017;27(5):1831-1839. doi: 10.1007/s00330-016-4540-y
- Wang X, Sun J, Zhang W, et al. Use of radiomics to extract splenic features to predict prognosis of patients with gastric cancer. Eur J Surg Oncol. 2020;46(10 Pt A):1932-1940. doi: 10.1016/j.ejso.2020.06.021
- Wang S, Feng C, Dong D, et al. Preoperative computed tomography-guided disease-free survival prediction in gastric cancer: A multicenter radiomics study. Med Phys. 2020;47(10):4862-4871. doi: 10.1002/mp.14350
- Li W, Zhang L, Tian C, et al. Prognostic value of computedtomography radiomics features in patients with gastric cancer following curative resection. Eur Radiol. 2019;29(6):3079- 3089. doi: 10.1007/s00330-018-5861-9
- Li Z, Zhang D, Dai Y, et al. Computed tomography-based radiomics for prediction of neoadjuvant chemotherapy outcomes in locally advanced gastric cancer: A pilot study. Chin J Cancer Res. 2018;30(4):406-414. doi: 10.21147/j.issn.1000-9604.2018.04.03
- Sun KY, Hu HT, Chen SL, et al. CT-based radiomics scores predict response to neoadjuvant chemotherapy and survival in patients with gastric cancer. BMC Cancer. 2020;20(1):468. doi: 10.1186/s12885-020-06970-7
- Mazzei MA, Di Giacomo L, Bagnacci G, et al. Delta-radiomics and response to neoadjuvant treatment in locally advanced gastric cancer-a multicenter study of GIRCG (Italian Research Group for Gastric Cancer). Quant Imaging Med Surg. 2021;11(6):2376-2387. doi: 10.21037/qims-20-683
- Xu Q, Sun Z, Li X, et al. Advanced gastric cancer: CT radiomics prediction and early detection of downstaging with neoadjuvant chemotherapy. Eur Radiol. 2021;31(11):8765- 8774. doi: 10.1007/s00330-021-07962-2
- Chen Y, Wei K, Liu D, et al. A machine learning model for predicting a major response to neoadjuvant chemotherapy in advanced gastric cancer. Front Oncol. 2021;11:675458. doi: 10.3389/fonc.2021.675458
- Chen Y, Yuan F, Wang L, et al. Evaluation of dual-energy CT derived radiomics signatures in predicting outcomes in patients with advanced gastric cancer after neoadjuvant chemotherapy. Eur J Surg Oncol. 2022;48(2):339-347. doi: 10.1016/j.ejso.2021.07.014
- Shin J, Lim JS, Huh YM, et al. A radiomics-based model for predicting prognosis of locally advanced gastric cancer in the preoperative setting. Sci Rep. 2021;11(1):1879. doi: 10.1038/s41598-021-81408-z
- Tan Z. Recent advances in the surgical treatment of advanced gastric cancer: A review. Med Sci Monit. 2019;25:3537-3541. doi: 10.12659/MSM.916475
- Klaassen R, Larue RTH, Mearadji B, et al. Feasibility of CT radiomics to predict treatment response of individual liver metastases in esophagogastric cancer patients. PLoS One. 2018;13(11):e0207362. doi: 10.1371/journal.pone.0207362
- Li J, Zhang C, Wei J, et al. Intratumoral and peritumoral radiomics of contrast-enhanced CT for prediction of disease-free survival and chemotherapy response in stage II/III gastric cancer. Front Oncol. 2020;10:552270. doi: 10.3389/fonc.2020.552270
- Wang N, Wang X, Li W, et al. Contrast-enhanced CT parameters of gastric adenocarcinoma: Can radiomic features be surrogate biomarkers for HER2 over-expression status? Cancer Manag Res. 2020;12:1211-1219. doi: 10.2147/CMAR.S230138
- Wang Y, Yu Y, Han W, et al. CT radiomics for distinction of human epidermal growth factor receptor 2 negative gastric cancer. Acad Radiol. 2021;28(3):e86-e92. doi: 10.1016/j.acra.2020.02.018
- Binnewies M, Roberts EW, Kersten K, et al. Understanding the tumor immune microenvironment (TIME) for effective therapy. Nat Med. 2018;24(5):541-550. doi: 10.1038/s41591-018-0014-x
- Jiang Y, Wang H, Wu J, et al. Noninvasive imaging evaluation of tumor immune microenvironment to predict outcomes in gastric cancer. Ann Oncol. 2020;31(6):760-768. doi: 10.1016/j.annonc.2020.03.295
- Lin JX, Lin JP, Weng Y, et al. Radiographical evaluation of tumor immunosuppressive microenvironment and treatment outcomes in gastric cancer: A retrospective, multicohort study. Ann Surg Oncol. 2022;29(8):5022-5033. doi: 10.1245/s10434-022-11499-z
- Gao X, Ma T, Bai S, et al. A CT-based radiomics signature for evaluating tumor infiltrating Treg cells and outcome prediction of gastric cancer. Ann Transl Med. 2020;8(7):469. doi: 10.21037/atm.2020.03.114
- Li J, Zhang C, Guo H, et al. Non-invasive measurement of tumor immune microenvironment and prediction of survival and chemotherapeutic benefits from 18F fluorodeoxyglucose PET/CT images in gastric cancer. Front Immunol. 2022;13:1019386. doi: 10.3389/fimmu.2022.1019386
- Avanzo M, Wei L, Stancanello J, et al. Machine and deep learning methods for radiomics. Med Phys. 2020;47(5):e185-e202. doi: 10.1002/mp.13678
- Zhang L, Dong D, Zhang W, et al. A deep learning risk prediction model for overall survival in patients with gastric cancer: A multicenter study. Radiother Oncol. 2020;150:73-80. doi: 10.1016/j.radonc.2020.06.010
- Tan JW, Wang L, Chen Y, et al. Predicting chemotherapeutic response for far-advanced gastric cancer by radiomics with deep learning semi-automatic segmentation. J Cancer. 2020;11(24):7224-7236. doi: 10.7150/jca.46704
- Dong D, Fang MJ, Tang L, et al. Deep learning radiomic nomogram can predict the number of lymph node metastasis in locally advanced gastric cancer: An international multicenter study. Ann Oncol. 2020;31(7):912-920. doi: 10.1016/j.annonc.2020.04.003
- Berlth F, Bollschweiler E, Drebber U, Hoelscher AH, Moenig S. Pathohistological classification systems in gastric cancer: Diagnostic relevance and prognostic value. World J Gastroenterol. 2014;20(19):5679-5684. doi: 10.3748/wjg.v20.i19.5679
- Cann C, Ciombor KK. Systemic therapy for gastric cancer: Perioperative strategies and beyond. J Surg Oncol. 2022;125(7):1151-1160. doi: 10.1002/jso.26834
- Zhang N, Fei Q, Gu J, Yin L, He X. Progress of preoperative and postoperative radiotherapy in gastric cancer. World J Surg Oncol. 2018;16(1):187. doi: 10.1186/s12957-018-1490-7
- Marcus C, Subramaniam RM. PET/computed tomography and precision medicine: Gastric cancer. PET Clin. 2017;12(4):437-447. doi: 10.1016/j.cpet.2017.05.004
- Kim GH. Systematic endoscopic approach to early gastric cancer in clinical practice. Gut Liver. 2021;15(6):811-817. doi: 10.5009/gnl20318
- Berenguer R, Pastor-Juan MDR, Canales-Vázquez J, et al. Radiomics of CT features may be nonreproducible and redundant: Influence of CT acquisition parameters. Radiology. 2018;288(2):407-415. doi: 10.1148/radiol.2018172361
- Wei L, Osman S, Hatt M, El Naqa I. Machine learning for radiomics-based multimodality and multiparametric modeling. Q J Nucl Med Mol Imaging. 2019;63(4):323-338. doi: 10.23736/S1824-4785.19.03213-8