Rheology-informed hierarchical machine learning model for the prediction of printing resolution in extrusion-based bioprinting
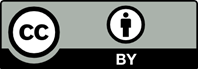
In this study, a rheology-informed hierarchical machine learning (RIHML) model was developed to improve the prediction accuracy of the printing resolution of constructs fabricated by extrusion-based bioprinting. Specifically, the RIHML model, as well as conventional models such as the concentration-dependent model and printing parameter-dependent model, was trained and tested using a small dataset of bioink properties and printing parameters. Interestingly, the results showed that the RIHML model exhibited the lowest error percentage in predicting the printing resolution for different printing parameters such as nozzle velocities and pressures, as well as for different concentrations of the bioink constituents. Besides, the RIHML model could predict the printing resolution with reasonably low errors even when using a new material added to the alginate-based bioink, which is a challenging task for conventional models. Overall, the results indicate that the RIHML model can be a useful tool to predict the printing resolution of extrusion-based bioprinting, and it is versatile and expandable compared to conventional models since the RIHML model can easily generalize and embrace new data.
- Ozbolat, Yu Y, 2013, Bioprinting toward organ fabrication: Challenges and future trends. IEEE Trans Biomed Eng, 60(3): 691–699. https://doi.org/10.1109/TBME.2013.2243912
- Murphy SV, Atala A, 2014, 3D bioprinting of tissues and organs. Nat Biotechnol, 32(8), 773–785. https://doi.org/10.1038/nbt.2958
- Sun W, Starly B, Daly AC, et al., 2020, The bioprinting roadmap. Biofabrication, 12(2): 022002. https://doi.org/10.1088/1758-5090/ab5158
- Daly AC, Prendergast ME, Hughes AJ, et al., 2021, Bioprinting for the biologist. Cell, 184(1): 18–32. https://doi.org/10.1016/j.cell.2020.12.002
- Lu D, Yang Y, Zhang P, et al., 2022, Development and application of three-dimensional bioprinting scaffold in the repair of spinal cord injury. Tissue Eng Regen Med, 19(6): 1113–1127. https://doi.org/10.1007/s13770-022-00465-1
- Tan B, Gan S, Wang X, et al., 2021, Applications of 3D bioprinting in tissue engineering: advantages, deficiencies, improvements, and future perspectives. J Mater Chem B, 9(27): 5385–5413. https://doi.org/10.1039/D1TB00172H
- Mandrycky C, Wang Z, Kim K, et al., 2016, 3D bioprinting for engineering complex tissues. Biotechnol Adv, 34(4): 422–434. https://doi.org/10.1016/j.biotechadv.2015.12.011
- Yilmaz B, Al Rashid A, Mou YA, et al., 2021, Bioprinting: A review of processes, materials and applications. Bioprinting, 23: e00148. https://doi.org/10.1016/j.bprint.2021.e00148
9. Mao X, Wang Z, 2022, Research progress of three-dimensional bioprinting artificial cardiac tissue. Tissue Eng Regen Med, 20: 1–9. https://doi.org/10.1007/s13770-022-00495-9
- Jacob GT, Passamai VE, Katz S, et al., 2022, Hydrogels for extrusion-based bioprinting: general considerations. Bioprinting, 27: e00212. https://doi.org/10.1016/j.bprint.2022.e00212
- Merceron TK, Burt M, Seol Y-J, et al., 2015, A 3D bioprinted complex structure for engineering the muscle–tendon unit. Biofabrication, 7(3): 035003. http://dx.doi.org/10.1088/1758-5090/7/3/035003
- Melchels FP, Dhert WJ, Hutmacher DW, et al., 2014, Development and characterisation of a new bioink for additive tissue manufacturing. J Mater Chem B, 2(16): 2282–2289. https://doi.org/10.1039/C3TB21280G
- Ozbolat IT, Hospodiuk M, 2016, Current advances and future perspectives in extrusion-based bioprinting. Biomaterials, 76: 321–343. https://doi.org/10.1016/j.biomaterials.2015.10.076
- Zhang YS, Haghiashtiani G, Hübscher T, et al., 2021, 3D extrusion bioprinting. Nat Rev Methods Primers, 1(1): 75. https://doi.org/10.1038/s43586-021-00073-8
- Ning L, Chen X, 2017, A brief review of extrusion‐based tissue scaffold bio‐printing. Biotechnol J, 12(8): 1600671. https://doi.org/10.1002/biot.201600671
- Xuan Z, Peng Q, Larsen T, et al., 2023, Tailoring hydrogel composition and stiffness to control smooth muscle cell differentiation in bioprinted constructs. Tissue Eng Regen Med, 20(2): 199–212. https://doi.org/10.1007/s13770-022-00500-1
- Willson K, Ke D, Kengla C, et al., 2020, Extrusion-based bioprinting: Current standards and relevancy for human-sized tissue fabrication. Methods Mol Biol, 2140: 65–92. https://doi.org/10.1007/978-1-0716-0520-2
- Hölzl K, Lin S, Tytgat L, et al., 2016, Bioink properties before, during and after 3D bioprinting. Biofabrication, 8(3): 032002. http://dx.doi.org/10.1088/1758-5090/8/3/032002
- Chung JH, Naficy S, Yue Z, et al., 2013, Bio-ink properties and printability for extrusion printing living cells. Biomater Sci, 1(7): 763–773. https://doi.org/10.1039/C3BM00012E
- Jin Y, Chai W, Huang Y, 2017, Printability study of hydrogel solution extrusion in nanoclay yield-stress bath during printing-then-gelation biofabrication. Mater Sci Eng C, 80: 313–325. https://doi.org/10.1016/j.msec.2017.05.144
- Datta P, Barui A, Wu Y, et al., 2018, Essential steps in bioprinting: From pre-to post-bioprinting. Biotechnol Adv, 36(5): 1481–1504. https://doi.org/10.1016/j.biotechadv.2018.06.003
- Sarker M, Naghieh S, McInnes AD, et al., 2019, Bio-fabrication of peptide-modified alginate scaffolds: Printability, mechanical stability and neurite outgrowth assessments. Bioprinting, 14: e00045. https://doi.org/10.1016/j.bprint.2019.e00045
- Gillispie G, Prim P, Copus J, et al., 2020, Assessment methodologies for extrusion-based bioink printability. Biofabrication, 12(2): 022003. https://doi.org/10.1088/1758-5090/ab6f0d
- Schwab A, Levato R, D’Este M, et al., 2020, Printability and shape fidelity of bioinks in 3D bioprinting. Chem Rev, 120(19): 11028–11055. https://doi.org/10.1021/acs.chemrev.0c00084
- Malekpour A, Chen X, 2022, Printability and cell viability in extrusion-based bioprinting from experimental, computational, and machine learning views. J Funct Biomater, 13(2): 40. https://doi.org/10.3390/jfb13020040
- Ramesh S, Harrysson OL, Rao PK, et al., 2021, Extrusion bioprinting: Recent progress, challenges, and future opportunities. Bioprinting, 21: e00116. https://doi.org/10.1016/j.bprint.2020.e00116
- Kang K, Hockaday L, Butcher J, 2013, Quantitative optimization of solid freeform deposition of aqueous hydrogels. Biofabrication, 5(3): 035001. http://dx.doi.org/10.1088/1758-5082/5/3/035001
- Tian S, Zhao H, Lewinski N, 2021, Key parameters and applications of extrusion-based bioprinting. Bioprinting, 23: e00156. https://doi.org/10.1016/j.bprint.2021.e00156
- Naghieh S, Chen X, 2021, Printability–A key issue in extrusion-based bioprinting. J Pharm Anal, 11(5): 564–579. https://doi.org/10.1016/j.jpha.2021.02.001
- Fu Z, Naghieh S, Xu C, et al., 2021, Printability in extrusion bioprinting. Biofabrication, 13(3): 033001. https://doi.org/10.1088/1758-5090/abe7ab
- Lee H, Yoo JM, Ponnusamy NK, et al., 2022, 3D-printed hydroxyapatite/gelatin bone scaffolds reinforced with graphene oxide: Optimized fabrication and mechanical characterization. Ceram Int, 48(7): 10155–10163. https://doi.org/10.1016/j.ceramint.2021.12.227
- Kim MH, Nam SY, 2020, Assessment of coaxial printability for extrusion-based bioprinting of alginate-based tubular constructs. Bioprinting, 20: e00092. https://doi.org/10.1016/j.bprint.2020.e00092
- Song K, Zhang D, Yin J, et al., 2021, Computational study of extrusion bioprinting with jammed gelatin microgel-based composite ink. Addit Manuf, 41: 101963. https://doi.org/10.1016/j.addma.2021.101963
- Leppiniemi J, Lahtinen P, Paajanen A, et al., 2017, 3D-printable bioactivated nanocellulose–alginate hydrogels. ACS Appl Mater Interfaces, 9(26): 21959–21970. https://doi.org/10.1021/acsami.7b02756
- Kim MH, Lee YW, Jung W-K, et al., 2019, Enhanced rheological behaviors of alginate hydrogels with carrageenan for extrusion-based bioprinting. J Mech Behav Biomed Mater, 98: 187–194. https://doi.org/10.1016/j.jmbbm.2019.06.014
- Göhl J, Markstedt K, Mark A, et al., 2018, Simulations of 3D bioprinting: Predicting bioprintability of nanofibrillar inks. Biofabrication, 10(3): 034105. https://doi.org/10.1088/1758-5090/aac872
- Bonatti AF, Chiesa I, Vozzi G, et al., 2021, Open-source CAD-CAM simulator of the extrusion-based bioprinting process. Bioprinting, 24: e00172. https://doi.org/10.1016/j.bprint.2021.e00172
- Suntornnond R, Tan EYS, An J, et al., 2016, A mathematical model on the resolution of extrusion bioprinting for the development of new bioinks. Materials, 9(9): 756. https://doi.org/10.3390/ma9090756
- Bonatti AF, Vozzi G, Chua CK, et al., A deep learning approach for error detection and quantification in extrusion-based bioprinting. Mater Today: Proc, 70: 131–135. https://doi.org/10.1016/j.matpr.2022.09.006
- Ruberu K, Senadeera M, Rana S, et al., 2021, Coupling machine learning with 3D bioprinting to fast track optimisation of extrusion printing. Appl Mater Today, 22: 100914. https://doi.org/10.1016/j.apmt.2020.100914
- Lee J, Oh SJ, An SH, et al., 2020, Machine learning-based design strategy for 3D printable bioink: Elastic modulus and yield stress determine printability. Biofabrication, 12(3): 035018. https://doi.org/10.1088/1758-5090/ab8707
- Conev A, Litsa EE, Perez MR, et al., 2020, Machine learning-guided three-dimensional printing of tissue engineering scaffolds. Tissue Eng Part A, 26(23–24): 1359–1368. https://doi.org/10.1089/ten.tea.2020.0191
- Fu Z, Angeline V, Sun W, 2021, Evaluation of printing parameters on 3D extrusion printing of pluronic hydrogels and machine learning guided parameter recommendation. Int J Bioprint, 7(4): 179–189. https://doi.org/10.18063%2Fijb.v7i4.434
- Bone JM, Childs CM, Menon A, et al., 2020, Hierarchical machine learning for high-fidelity 3D printed biopolymers. ACS Biomater Sci Eng, 6(12): 7021–7031. https://doi.org/10.1021/acsbiomaterials.0c00755
- Menon A, Póczos B, Feinberg AW, et al., 2019, Optimization of silicone 3D printing with hierarchical machine learning. 3D Print Addit Manuf, 6(4): 181–189. https://doi.org/10.1089/3dp.2018.0088
- Jin Z, Zhang Z, Shao X, et al., 2021, Monitoring anomalies in 3D bioprinting with deep neural networks. ACS Biomater Sci Eng, 9(7): 3945–3952. https://doi.org/10.1021/acsbiomaterials.0c01761
- Mancha Sánchez E, Gómez-Blanco JC, López Nieto, et al., 2020, Hydrogels for bioprinting: A systematic review of hydrogels synthesis, bioprinting parameters, and bioprinted structures behavior. Front Bioeng Biotechnol, 8: 776. https://doi.org/10.3389/fbioe.2020.00776
- Zhang Z, Jin Y, Yin J, et al., 2018, Evaluation of bioink printability for bioprinting applications. Appl Phys Rev, 5(4): 041304. https://doi.org/10.1063/1.5053979
- Chimene D, Lennox KK, Kaunas RR, et al., 2016, Advanced bioinks for 3D printing: A materials science perspective. Annal Biomed Eng, 44(6): 2090–2102. https://doi.org/10.1007/s10439-016-1638-y
- Cooke ME, Rosenzweig DH, 2021, The rheology of direct and suspended extrusion bioprinting. APL Bioeng, 5(1): 011502. https://doi.org/10.1063/5.0031475
- Paxton N, Smolan W, Böck T, et al., 2017, Proposal to assess printability of bioinks for extrusion-based bioprinting and evaluation of rheological properties governing bioprintability. Biofabrication, 9(4): 044107. https://doi.org/10.1088/1758-5090/aa8dd8
- Ouyang L, Yao R, Zhao Y, et al., 2016, Effect of bioink properties on printability and cell viability for 3D bioplotting of embryonic stem cells. Biofabrication, 8(3): 035020. http://dx.doi.org/10.1088/1758-5090/8/3/035020
- Xu X, Jagota A, Peng S, et al., 2013, Gravity and surface tension effects on the shape change of soft materials. Langmuir, 29(27): 8665–8674. https://doi.org/10.1021/la400921h
- Gao T, Gillispie GJ, Copus JS, et al., 2018, Optimization of gelatin–alginate composite bioink printability using rheological parameters: A systematic approach. Biofabrication, 10(3): 034106. https://doi.org/10.1088/1758-5090/aacdc7
- Yu C, Jiang J, 2020, A perspective on using machine learning in 3D bioprinting. Int J Bioprint, 6(1): 4–11. https://doi.org/10.18063%2Fijb.v6i1.253
- Shin J, Lee Y, Li Z, et al., 2022, Optimized 3D bioprinting technology based on machine learning: A review of recent trends and advances. Micromachines, 13(3): 363. https://doi.org/10.3390/mi13030363
- Ng WL, Chan A, Ong YS, et al., 2020, Deep learning for fabrication and maturation of 3D bioprinted tissues and organs. Virtual Phys Prototyp, 15(3): 340–358. https://doi.org/10.1080/17452759.2020.1771741