Evaluation of Printing Parameters on 3D Extrusion Printing of Pluronic Hydrogels and Machine Learning Guided Parameter Recommendation
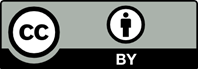
Bioprinting is an emerging technology for the construction of complex three-dimensional (3D) constructs used in various biomedical applications. One of the challenges in this field is the delicate manipulation of material properties and various disparate printing parameters to create structures with high fidelity. Understanding the effects of certain parameters and identifying optimal parameters for creating highly accurate structures are therefore a worthwhile subject to investigate. The objective of this study is to investigate high-impact print parameters on the printing printability and develop a preliminary machine learning model to optimize printing parameters. The results of this study will lead to an exploration of machine learning applications in bioprinting and to an improved understanding between 3D printing parameters and structural printability. Reported results include the effects of rheological property, nozzle gauge, nozzle temperature, path height, and ink composition on the printability of Pluronic F127. The developed Support Vector Machine model generated a process map to assist the selection of optimal printing parameters to yield high quality prints with high probability (>75%). Future work with more generalized machine learning models in bioprinting is also discussed in this article. The finding of this study provides a simple tool to improve printability of extrusion-based bioprinting with minimum experimentations.
1. Sun W, Starly B, Daly AC, et al., 2020, The Bioprinting Roadmap. Biofabrication, 12:022002. http://doi.org/10.1088/1758-5090/ab5158
2. Suntornnond R, Tan EY, An J, et al., 2017, A highly Printable and Biocompatible Hydrogel Composite for Direct Printing of Soft and Perfusable Vasculature-Like Structures. Sci Rep, 7:16902. https://doi.org/10.1038/s41598-017-17198-0
3. Kolesky DB, Truby RL, Gladman AS, et al., 2014, 3D Bioprinting of Vascularized, Heterogeneous Cell-Laden Tissue Constructs. Adv Mater, 26:3124–30. https://doi.org/10.1002/adma.201305506
4. An J, Teoh JE, Suntornnond R, et al., 2015, Design and 3D Printing of Scaffolds and Tissues. Engineering, 1:261–8. http://doi.org/10.15302/j-eng-2015061
5. Jessop ZM, Al-Sabah A, Gardiner MD, et al., 2017, 3D Bioprinting for Reconstructive Surgery: Principles, Applications and Challenges. J Plast Reconstr Aesthet Surg, 70:1155–70. https://doi.org/10.1016/j.bjps.2017.06.001
6. Kondiah PJ, Kondiah PP, Choonara YE, et al., 2020, A 3D Bioprinted Pseudo-Bone Drug Delivery Scaffold for Bone Tissue Engineering. Pharmaceutics, 12:166. https://doi.org/10.3390/pharmaceutics12020166
7. Ma X, Liu J, Zhu W, et al., 2018, 3D Bioprinting of Functional Tissue Models for Personalized Drug Screening and In Vitro Disease Modeling. Adv Drug Deliv Rev, 132:235–51. https://doi.org/10.1016/j.addr.2018.06.011
8. Ng WL, Lee JM, Zhou M, et al., 2020, Vat Polymerization-Based Bioprinting-Process, Materials, Applications and Regulatory Challenges. Biofabrication, 12:022001. https://doi.org/10.1088/1758-5090/ab6034
9. Lee JM, Sing SL, Zhou M, et al., 2018, 3D Bioprinting Processes: A Perspective on Classification and Terminology. Int J Bioprint, 4:151. https://doi.org/10.18063/ijb.v4i2.151
10. Ouyang L, Yao R, Zhao Y, et al., 2016, Effect of Bioink Properties on Printability and Cell Viability for 3D Bioplotting of Embryonic Stem Cells. Biofabrication, 8:035020. https://doi.org/10.1088/1758-5090/8/3/035020
11. Suntornnond R, An J, Chua CK, 2017, Bioprinting of Thermoresponsive Hydrogels for Next Generation Tissue Engineering: A Review. Macromol Mater Eng, 302:1600266. https://doi.org/10.1002/mame.201600266
12. Malda J, Visser J, Melchels FP, et al., 2013, 25th Anniversary Article: Engineering Hydrogels for Biofabrication. Adv Mater, 25:5011–28. https://doi.org/10.1002/adma.201302042
13. Hospodiuk M, Dey M, Sosnoski D, et al., 2017, The Bioink: A Comprehensive Review on Bioprintable Materials. Biotechnol Adv, 35:217–39. https://doi.org/10.1016/j.biotechadv.2016.12.006
14. Gungor-Ozkerim PS, Inci I, Zhang YS, et al., 2018, Bioinks for 3D Bioprinting: An Overview. Biomater Sci, 6:915–46. https://doi.org/10.1039/c7bm00765e
15. Fu Z, Naghieh S, Xu C, et al., 2021, Printability in Extrusion Bioprinting. Biofabrication, 3:033001. https://doi.org/10.1088/1758-5090/abe7ab
16. Gioffredi E, Boffito M, Calzone S, et al., 2016, Pluronic F127 Hydrogel Characterization and Biofabrication in Cellularized Constructs for Tissue Engineering Applications. Procedia CIRP, 49:125–32. https://doi.org/10.1016/j.procir.2015.11.001
17. Kang KH, Hockaday LA, Butcher JT, 2013, Quantitative Optimization of Solid Freeform Deposition of Aqueous Hydrogels. Biofabrication, 5:035001. https://doi.org/10.1088/1758-5082/5/3/035001
18. Soltan N, Ning L, Mohabatpour F, et al., 2019, Printability and Cell Viability in Bioprinting Alginate Dialdehyde-Gelatin Scaffolds. ACS Biomater Sci Eng, 5:2976–87. https://doi.org/10.1021/acsbiomaterials.9b00167
19. Naghieh S, Sarker MD, Sharma NK, et al., 2019, Printability of 3D Printed Hydrogel Scaffolds: Influence of Hydrogel Composition and Printing Parameters. Appl Sci, 10:292. https://doi.org/10.3390/app10010292
20. Paxton N, Smolan W, Bock T, et al., 2017, Proposal to Assess Printability of Bioinks for Extrusion-Based Bioprinting and Evaluation of Rheological Properties Governing Bioprintability. Biofabrication, 9:044107. https://doi.org/10.1088/1758-5090/aa8dd8
21. Yu C, Jiang J, 2020, A Perspective on Using Machine Learning in 3D Bioprinting. Int J Bioprint, 6:253. http://doi.org/10.18063/ijb.v6i1.253
22. Conev A, Litsa EE, Perez MR, et al., 2020, Machine Learning-Guided Three-Dimensional Printing of Tissue Engineering Scaffolds. Tissue Eng Part A, 26:1359–68. https://doi.org/10.1089/ten.tea.2020.0191
23. Menon A, Póczos B, Feinberg AW, et al., 2019, Optimization of Silicone 3D Printing with Hierarchical Machine Learning. 3D Print Addit Manuf, 6:181–9. https://doi.org/10.1089/3dp.2018.0088
24. Ruberu K, Senadeera M, Rana S, et al., 2021, Coupling Machine Learning with 3D Bioprinting to Fast Track Optimisation of Extrusion Printing. Appl Mater Today, 22:100914. https://doi.org/10.1016/j.apmt.2020.100914
25. Holzl K, Lin SM, Tytgat L, et al., 2016, Bioink Properties Before, during and after 3D Bioprinting. Biofabrication, 8:032002. https://doi.org/10.1088/1758-5090/8/3/032002
26. Suntornnond R, Tan EY, An J, et al., 2016, A Mathematical Model on the Resolution of Extrusion Bioprinting for the Development of New Bioinks. Materials, 9:756. https://doi.org/10.3390/ma9090756
27. Aoyagi K, Wang H, Sudo H, et al., 2019, Simple Method to Construct Process Maps for Additive Manufacturing using a Support Vector Machine. Addit Manuf, 27:353–62. https://doi.org/10.1016/j.addma.2019.03.013
28. Fang KT, Lin DK, Winker P, et al., 2000, Uniform Design: Theory and Application. Technometrics, 42:237–48. https://doi.org/10.1080/00401706.2000.10486045
29. Boser BE, Guyon IM, Vapnik VN, 1992, A Training Algorithm for Optimal Margin Classifiers. In: Proceedings of the Fifth Annual Workshop on Computational Learning Theory, p144–52. https://doi.org/10.1145/130385.130401
30. Chang CC, Lin CJ, 2011, LIBSVM: A Library for Support Vector Machines. ACM Trans Intell Syst Technol, 2:1–27. https://doi.org/10.1145/1961189.1961199
31. Wu TF, Lin CJ, Weng RC, 2004, Probability Estimates for Multi-Class Classification by Pairwise Coupling. J Mach Learn Res, 5:975–1005. https://doi.org/10.5555/1005332.1016791
32. Matanović MR, Kristl J, Grabnar PA, 2014, Thermoresponsive Polymers: Insights Into Decisive Hydrogel Characteristics, Mechanisms of Gelation, and Promising Biomedical Applications. Int J Pharm, 472:262–75. https://doi.org/10.1016/j.ijpharm.2014.06.029
33. Khalil S, Sun W, 2007, Biopolymer Deposition for Freeform Fabrication of Hydrogel Tissue Constructs. Mater Sci Eng C, 27:469–78. https://doi.org/10.1016/j.msec.2006.05.02