Application of Machine Learning in 3D Bioprinting: Focus on Development of Big Data and Digital Twin
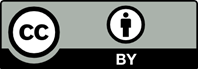
The application of machine learning (ML) in bioprinting has attracted considerable attention recently. Many have focused on the benefits and potential of ML, but a clear overview of how ML shapes the future of three-dimensional (3D) bioprinting is still lacking. Here, it is proposed that two missing links, Big Data and Digital Twin, are the key to articulate the vision of future 3D bioprinting. Creating training databases from Big Data curation and building digital twins of human organs with cellular resolution and properties are the most important and urgent challenges. With these missing links, it is envisioned that future 3D bioprinting will become more digital and in silico, and eventually strike a balance between virtual and physical experiments toward the most efficient utilization of bioprinting resources. Furthermore, the virtual component of bioprinting and biofabrication, namely, digital bioprinting, will become a new growth point for digital industry and information technology in future.
1. Yu C, Jiang J, 2020, A Perspective on Using Machine Learning in 3D Bioprinting. Int J Bioprinting, 6:95.https://doi.org/10.18063/ijb.v6i1.253
2. Ng WL, Chan A, Ong YS, et al., 2020, Deep Learning for Fabrication and Maturation of 3D Bioprinted Tissues and Organs. Virtual Phys Prototyp, 15:340–58.
3. Meng L, McWilliams B, Jarosinski W, et al., 2020, Machine Learning in Additive Manufacturing: A Review. JOM, 72:1–15.
4. Goh G, Sing S, Yeong W, 2020, A Review on Machine Learning in 3D Printing: Applications, Potential, and Challenges. Artif Intell Rev, 54:63–94. https://doi.org/10.1007/s10462-020-09876-9
5. Hamid OA, Eltaher HM, Sottile V, et al., 2020, 3D Bioprinting of a Stem Cell-laden, Multi-material Tubular Composite: An Approach for Spinal Cord Repair. Mater Sci Eng C, 2020:111707. https://doi.org/10.1016/j.msec.2020.111707
6. Lee JM, Sing SL, Yeong WY, 2020, Bioprinting of Multimaterials with Computer-aided Design/Computer-aided Manufacturing. Int J Bioprint, 2020:245. https://doi.org/10.18063/ijb.v6i1.245
7. Geris L, Papantoniou I, 2019, The Third Era of Tissue Engineering: Reversing the Innovation Drivers. Tissue Eng Part A, 25:821–6. https://doi.org/10.1089/ten.tea.2019.0064
8. Campos DF, De Laporte L, 2020, Digitally Fabricated and Naturally Augmented In Vitro Tissues. Adv Healthc Mater, 2020:2001253. https://doi.org/10.1002/adhm.202001253
9. Barnard AS, 2014, In silico veritas. ACS Nano, 8:6520–5.
10. Ruberu K, Senadeera M, Rana S, et al., Coupling Machine Learning with 3D Bioprinting to Fast Track Optimisation of Extrusion Printing. Appl Mater Today, 22:100914. https://doi.org/10.1016/j.apmt.2020.100914
11. Zhang Z, Jin Y, Xu C, et al., 2018, Evaluation of Bioink Printability for Bioprinting Applications. Appl Phys Rev, 5:041304.
12. Schwab A, Levato R, D’Este M, et al., 2020, Printability and Shape Fidelity of Bioinks in 3D Bioprinting. Chem Rev, 120:11028–55. https://doi.org/10.1021/acs.chemrev.0c00084
13. Arai F, Stumpf PS, Ikushima YM, et al., 2020, Machine Learning of Hematopoietic Stem Cell Divisions from Paired Daughter Cell Expression Profiles Reveals Effects of aging on Self-Renewal. Cell Syst, 11:640–52. https://doi.org/10.1016/j.cels.2020.11.004
14. Cilloni D, Petiti J, Campia V, et al., 2020, Transplantation Induces Profound Changes in the Transcriptional Asset of Hematopoietic Stem Cells: Identification of Specific Signatures Using Machine Learning Techniques. J Clin Med, 9:1670. https://doi.org/10.3390/jcm9061670
15. Rivenson Y, Wang H, Wei Z, et al., 2019, Virtual Histological Staining of Unlabelled Tissue-Autofluorescence Images via Deep Learning. Nat Biomed Eng, 3:466. https://doi.org/10.1038/s41551-019-0362-y
16. Zhang Y, de Haan K, Rivenson Y, et al., 2020, Digital Synthesis of Histological Stains Using Micro-Structured and Multiplexed Virtual Staining of Label-free Tissue. Light, 9:1–13. https://doi.org/10.1038/s41377-020-0315-y
17. Rivenson Y, de Haan K, Wallace A, et al., 2020, Emerging Advances to Transform Histopathology Using Virtual Staining. BME Front, 2020:9647163. https://doi.org/10.34133/2020/9647163
18. MacArthur BD, Stumpf PS, Oreffo RO, 2020, From Mathematical Modeling and Machine Learning to Clinical Reality. In: Lanza R, Langer R, Vacanti J, editors. Principles of Tissue Engineering. 5th ed., Ch. 2. Academic Press, Cambridge, Massachusetts, pp. 37–51. https://doi.org/10.1016/b978-0-12-818422-6.00001-0
19. What is the Structure of Big Data? 2019. Available from: https://www.magnimindacademy.com/blog/whatis- the-structure-of-big-data#:~:text=Big%20data%20 structures%20can%20be,look%20at%20them%20in%20 detail. [Last accessed on 2020 Dec 25].
20. Yan X, Sedykh A, Wang W, et al., 2020, Construction of a Web-based Nanomaterial Database by Big Data Curation and Modeling Friendly Nanostructure Annotations. Nat Commun, 11:1–10. https://doi.org/10.1038/s41467-020-16413-3
21. Bergen KJ, Johnson PA, de Hoop MV, et al., 2019, Machine Learning for Data-driven Discovery in Solid Earth Geoscience. Science, 363:eaau0323. https://doi.org/10.1126/science.aau0323
22. Clauset A, Larremore DB, Sinatra R, 2017, Data-Driven Predictions in the Science of Science. Science, 355:477–80. https://doi.org/10.1126/science.aal4217
23. Consortium H, 2019, The Human Body at Cellular Resolution: The NIH Human Biomolecular Atlas Program. Nature, 574:187. https://doi.org/10.1038/s41586-019-1629-x
24. Hoehme S, Drasdo D, 2010, A Cell-based Simulation Software for Multi-Cellular Systems. Bioinformatics, 26:2641–2. https://doi.org/10.1093/bioinformatics/btq437
25. Rogers WJ, Meyer CH, Kramer CM, 2006, Technology Insight: In Vivo Cell Tracking by Use of MRI. Nat Clin Pract Cardiovasc Med, 3:554–62.
26. Sommer C, Gerlich DW, 2013, Machine Learning in Cell Biology-Teaching Computers to Recognize Phenotypes. J Cell Sci, 126:5529–39. https://doi.org/10.1242/jcs.123604
27. Daly AC, Prendergast ME, Hughes AJ, et al., 2021, Bioprinting for the Biologist. Cell, 184:18–32. https://doi.org/10.1016/j.cell.2020.12.002
28. Ng WL, Chua CK, Shen YF, 2019, Print me an Organ! Why we are not there yet. Prog Polym Sci, 97:101145. https://doi.org/10.1016/j.progpolymsci.2019.101145
29. Sacha GM, Varona P, 2013, Artificial Intelligence in Nanotechnology. Nanotechnology, 24:452002. https://doi.org/10.1088/0957-4484/24/45/452002
30. Hamel CM, Roach DJ, Long KN, et al., 2019, Machine learning Based Design of Active Composite Structures for 4D Printing. Smart Mater Struct, 28:065005. https://doi.org/10.1088/1361-665x/ab1439
31. An J, Chua CK, Mironov V, 2016, A Perspective on 4D Bioprinting. Int J Bioprint, 2:02003.
32. Jackson B, 2019, Aether to Develop Ai 3D Bioprinter in Agreement with Procter and Gamble. Available from: https://www.3dprintingindustry.com/news/aether-to-develop-ai-3dbioprinter-in-agreement-with-procter-gamble-149405. [Last accessed on 2020 Dec 20].
33. Rezende RA, Kasyanov V, Mironov V, et al., 2015, Organ Printing as an Information Technology. Proc Eng, 110:151–8.
34. Dernowsek JD, Rezende RA, Lopes da Silva JV, 2-17, The Role of Information Technology in the Future of 3D Biofabrication. J 3D Print Med, 1:63–74.