Artificial intelligence-driven material development for additive manufacturing: A critical review
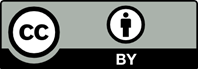
Additive manufacturing (AM) has revolutionized material fabrication by enabling the production of complex structures with enhanced design flexibility and material efficiency. However, the development of AM-specific materials remains a critical challenge due to the unique process characteristics of AM. Recent advancements in artificial intelligence (AI), for example, machine learning and deep learning, have emerged as powerful tools in accelerating material discovery, optimizing process parameters, and improving material performance for AM. This review provides a comprehensive overview of AI-driven material development for AM, focusing on metals, polymers, and bioinks/biomaterial inks. The discussion encompasses AI techniques applied to material development, including predictive modeling, generative algorithms, and intelligent optimization methods. Data collection and pre-processing methodologies for AI applications in AM are discussed. In addition, the applications of AI in material development in AM are also reviewed. Finally, the review highlights emerging trends, such as AI-driven high-throughput material screening, integration of AI with multiscale high-fidelity simulations, the use of digital twins for real-time process control, and active learning strategies for optimizing material compositions. By summarizing recent advancements and outlining future directions, this review provides insights into the evolving intersection of AI and AM, paving the way for more intelligent and efficient material development in the next generation of manufacturing.
- Tan C, Li R, Su J, et al. Review on field assisted metal additive manufacturing. Int J Mach Tools Manuf. 2023;189:104032. doi: 10.1016/j.ijmachtools.2023.104032
- Su J, Jiang F, Teng J, et al. Recent innovations in laser additive manufacturing of titanium alloys. Int J Extreme Manuf. 2024;6(3):032001. doi: 10.1088/2631-7990/ad2545
- Liu Y, Sing SL. A review of advances in additive manufacturing and the integration of high-performance polymers, alloys, and their composites. Mater Sci Addit Manuf. 2023;2(3):1587. doi: 10.36922/msam.1587
- Su J, Ng WL, An J, Yeong WY, Chua CK, Sing SL. Achieving sustainability by additive manufacturing: A state-of-the-art review and perspectives. Virtual Phys Prototyp. 2024;19(1):e2438899. doi: 10.1080/17452759.2024.2438899
- Mondal S, Shubhra Goswami S. Machine learning techniques for quality assurance in additive manufacturing processes. Int J AI Mater Des. 2024;1(2):21. doi: 10.36922/ijamd.3455
- Su JL, Jiang FL, Teng J, et al. Laser additive manufacturing of titanium alloys: Process, materials and post-processing. Rare Met. 2024;43(12):6288-6328. doi: 10.1007/s12598-024-02685-x
- Seo E, Sung H, Jeon H, et al. Laser powder bed fusion for AI assisted digital metal components. Virtual Phys Prototyp. 2022;17(4):806-820. doi: 10.1080/17452759.2022.2068804
- Kim T, Kim JG, Park S, et al. Virtual surface morphology generation of Ti-6Al-4V directed energy deposition via conditional generative adversarial network. Virtual Phys Prototyp. 2023;18(1):e2124921. doi: 10.1080/17452759.2022.2124921
- Zhang J, Yin C, Xu Y, Sing SL. Machine learning applications for quality improvement in laser powder bed fusion: A state-of-the-art review. Int J AI Mater Des. 2024;1(1):26. doi: 10.36922/ijamd.2301
- Jin L, Zhai X, Wang K, et al. Big data, machine learning, and digital twin assisted additive manufacturing: A review. Mater Des. 2024;244:113086. doi: 10.1016/j.matdes.2024.113086
- Al Rashid A, Ahmed W, Khalid MY, Koç M. Vat photopolymerization of polymers and polymer composites: Processes and applications. Addit Manuf. 2021;47:102279. doi: 10.1016/j.addma.2021.102279
- Pagac M, Hajnys J, Ma QP, et al. A review of vat photopolymerization technology: Materials, applications, challenges, and future trends of 3D printing. Polymers. 2021;13(4):598. doi: 10.3390/polym13040598
- Gibson I, Rosen D, Stucker B, Khorasani M. Additive Manufacturing Technologies. Berlin: Springer International Publishing; 2021. doi: 10.1007/978-3-030-56127-7
- Elkaseer A, Chen KJ, Janhsen JC, Refle O, Hagenmeyer V, Scholz SG. Material jetting for advanced applications: A state-of-the-art review, gaps and future directions. Addit Manuf. 2022;60:103270. doi: 10.1016/j.addma.2022.103270
- Chou WH, Gamboa A, Morales JO. Inkjet printing of small molecules, biologics, and nanoparticles. Int J Pharm. 2021;600:120462. doi: 10.1016/j.ijpharm.2021.120462
- Gülcan O, Günaydın K, Tamer A. The state of the art of material jetting-a critical review. Polymers (Basel). 2021;13(16):2829. doi: 10.3390/polym13162829
- Sachs E, Cima M, Williams P, Brancazio D, Cornie J. Three dimensional printing: Rapid tooling and prototypes directly from a CAD model. J Eng Ind. 1992;114(4):481-488. doi: 10.1115/1.2900701
- Naitoh M, Kubota Y, Katsumata A, Ohsaki C, Ariji E. Dimensional accuracy of a binder jet model produced from computerized tomography data for dental implants. J Oral Implantol. 2006;32(6):273-276. doi: 10.1563/1548-1336(2006)32[273:DAOABJ]2.0.CO;2
- Deng C, Kang J, Shangguan H, Hu Y, Huang T, Liu Z. Effects of hollow structures in sand mold manufactured using 3D printing technology. J Mater Process Technol. 2018;255:516-523.
- Holland S, Foster T, Tuck C. Creation of food structures through binder jetting. In: Fundamentals of 3D Food Printing and Applications. Elsevier; 2019. p. 257-288. Available from: https://www.sciencedirect.com/science/article/pii/ B9780128145647000092 [Last accessed on 2024 Dec 16].
- Su J, Li Q, Teng J, et al. Programmable mechanical properties of additively manufactured novel steel. Int J Extreme Manuf. 2025;7(1):015001. doi: 10.1088/2631-7990/ad88bc
- Priyadarshini BM, Kok WK, Dikshit V, Feng S, Li KHH, Zhang Y. 3D printing biocompatible materials with Multi Jet Fusion for bioreactor applications. Int J Bioprinting. 2022;9(1):623. doi: 10.18063/ijb.v9i1.623
- Singh R, Gupta A, Tripathi O, et al. Powder bed fusion process in additive manufacturing: An overview. Mater Today Proc. 2020;26:3058-3070. doi: 10.1016/j.matpr.2020.02.635
- Gharraei R, Bergstrom DJ, Chen X. Extrusion bioprinting from a fluid mechanics perspective. Int J Bioprinting. 2024;10(6):3973. doi: 10.36922/ijb.3973
- Svetlizky D, Das M, Zheng B, et al. Directed energy deposition (DED) additive manufacturing: Physical characteristics, defects, challenges and applications. Mater Today. 2021;49:271-295. doi: 10.1016/j.mattod.2021.03.020
- Kumar A, Dixit AR, Sreenivasa S. Mechanical properties of additively manufactured polymeric composites using sheet lamination technique and fused deposition modeling: A review. Polym Adv Technol. 2024;35(4):e6396. doi: 10.1002/pat.6396
- Tepylo N, Huang X, Patnaik PC. Laser‐based additive manufacturing technologies for aerospace applications. Adv Eng Mater. 2019;21(11):1900617. doi: 10.1002/adem.201900617
- Hofstätter T, Pedersen DB, Tosello G, Hansen HN. State-of-the-art of fiber-reinforced polymers in additive manufacturing technologies. J Reinf Plast Compos. 2017;36(15):1061-1073. doi: 10.1177/0731684417695648
- Kussmaul R, Biedermann M, Pappas GA, et al. Individualized lightweight structures for biomedical applications using additive manufacturing and carbon fiber patched composites. Des Sci. 2019;5:e20.
- Li Y, Feng Z, Huang L, et al. Additive manufacturing high performance graphene-based composites: A review. Compos Part Appl Sci Manuf. 2019;124:105483.
- Wang B, Tao F, Fang X, Liu C, Liu Y, Freiheit T. Smart manufacturing and intelligent manufacturing: A comparative review. Engineering. 2021;7(6):738-757.
- Xiao S, Li J, Wang Z, Chen Y, Tofighi S. Advancing additive manufacturing through machine learning techniques: A state-of-the-art review. Future Internet. 2024;16:419.
- Dang L, He X, Tang D, Li Y, Wang T. A fatigue life prediction approach for laser-directed energy deposition titanium alloys by using support vector regression based on pore-induced failures. Int J Fatigue. 2022;159:106748.
- Ling J, Hutchinson M, Antono E, Paradiso S, Meredig B. High-dimensional materials and process optimization using data-driven experimental design with well-calibrated uncertainty estimates. Integr Mater Manuf Innov. 2017;6:207-217.
- Navarrete I, Fé-Perdomo IL, Ramos-Grez JA, Lopez M. Predicting the evolution of static yield stress with time of blended cement paste through a machine learning approach. Constr Build Mater. 2023;371:130632. doi: 10.1016/j.conbuildmat.2023.130632
- Khurana D, Koli A, Khatter K, Singh S. Natural language processing: State of the art, current trends and challenges. Multimed Tools Appl. 2023;82(3):3713-3744. doi: 10.1007/s11042-022-13428-4
- Gu GX, Chen CT, Richmond DJ, Buehler MJ. Bioinspired hierarchical composite design using machine learning: Simulation, additive manufacturing, and experiment. Mater Horiz. 2018;5(5):939-945. doi: 10.1039/C8MH00653A
- Li J, Yang Z, Qian G, Berto F. Machine learning based very-high-cycle fatigue life prediction of Ti-6Al-4V alloy fabricated by selective laser melting. Int J Fatigue. 2022;158:106764.
- Shen SC, Buehler MJ. Nature-inspired architected materials using unsupervised deep learning. Commun Eng. 2022;1(1):37.
- Lee SY, Byeon S, Kim HS, Jin H, Lee S. Deep learning-based phase prediction of high-entropy alloys: Optimization, generation, and explanation. Mater Des. 2021;197:109260. doi: 10.1016/j.matdes.2020.109260
- Buehler MJ. MechGPT, a language-based strategy for mechanics and materials modeling that connects knowledge across scales, disciplines, and modalities. Appl Mech Rev. 2024;76(2):021001. doi: 10.1115/1.4063843
- Nazar S, Yang J, Faisal Javed M, Khan K, Li L, Liu QF. An evolutionary machine learning-based model to estimate the rheological parameters of fresh concrete. Structures. 2023;48:1670-1683. doi: 10.1016/j.istruc.2023.01.019
- Hu M, Tan Q, Knibbe R, et al. Recent applications of machine learning in alloy design: A review. Mater Sci Eng R Rep. 2023;155:100746. doi: 10.1016/j.mser.2023.100746
- Curtarolo S, Setyawan W, Wang S, et al. AFLOWLIB. ORG: A distributed materials properties repository from high-throughput ab initio calculations. Comput Mater Sci. 2012;58:227-235. doi: 10.1016/j.commatsci.2012.02.002
- Gražulis S, Daškevič A, Merkys A, et al. Crystallography open database (COD): An open-access collection of crystal structures and platform for world-wide collaboration. Nucleic Acids Res. 2012;40(D1):D420-D427. doi: 10.1093/nar/gkr900
- Belsky A, Hellenbrandt M, Karen VL, Luksch P. New developments in the Inorganic Crystal Structure Database (ICSD): Accessibility in support of materials research and design. Acta Crystallogr B. 2002;58(3):364-369. doi: 10.1107/S0108768102006948
- Choudhary K, Garrity KF, Reid ACE, et al. The joint automated repository for various integrated simulations (JARVIS) for data-driven materials design. NPJ Comput Mater. 2020;6(1):173. doi: 10.1038/s41524-020-00440-1
- Talirz L, Kumbhar S, Passaro E, et al. Materials cloud, a platform for open computational science. Sci Data. 2020;7(1):299. doi: 10.1038/s41597-020-00637-5
- Draxl C, Scheffler M. NOMAD: The FAIR concept for big data-driven materials science. MRS Bull. 2018;43(9):676-682. doi: 10.1557/mrs.2018.208
- Tadmor EB, Elliott RS, Sethna JP, Miller RE, Becker CA. The potential of atomistic simulations and the knowledgebase of interatomic models. JOM. 2011;63(7):17. doi: 10.1007/s11837-011-0102-6
- Jain A, Ong SP, Hautier G, et al. Commentary: The materials project: A materials genome approach to accelerating materials innovation. APL Mater. 2013;1(1):011002. doi: 10.1063/1.4812323
- Saal JE, Kirklin S, Aykol M, Meredig B, Wolverton C. Materials design and discovery with high-throughput density functional theory: The open quantum materials database (OQMD). JOM. 2013;65(11):1501-1509. doi: 10.1007/s11837-013-0755-4
- Lakshminarayan K, Harp SA, Goldman R, Samad T. Imputation of Missing Data Using Machine learning Techniques. In: Simoudis E, Han J, Fayyad UM, editors. Proceedings - 2nd International Conference on Knowledge Discovery and Data Mining, KDD 1996. AAAI Press; 1996. p. 140-145.
- Mahato V, Chatterjee S, Nyabadza A, Caputo A, Brabazon D. Layer porosity in powder-bed fusion prediction using regression machine learning models and time-series features. Int J AI Mater Des. 2024;1(3):33-49. doi: 10.36922/ijamd.4812
- Montazeri M, Nassar AR, Dunbar AJ, Rao P. In-process monitoring of porosity in additive manufacturing using optical emission spectroscopy. IISE Trans. 2020;52(5):500-515. doi: 10.1080/24725854.2019.1659525
- Wasmer K, Le-Quang T, Meylan B, Shevchik S. A. In situ quality monitoring in AM using acoustic emission: A reinforcement learning approach. J Mater Eng Perform. 2019;28(2):666-672. doi: 10.1007/s11665-018-3690-2
- Jolliffe IT, Cadima J. Principal component analysis: A review and recent developments. Philos Trans R Soc Math Phys Eng Sci. 2016;374(2065):20150202. doi: 10.1098/rsta.2015.0202
- Tan C, Li Q, Yao X, et al. Machine learning customized novel material for energy‐efficient 4D printing. Adv Sci. 2023;10(10):2206607. doi: 10.1002/advs.202206607
- Zhang M, Sun CN, Zhang X, et al. High cycle fatigue life prediction of laser additive manufactured stainless steel: A machine learning approach. Int J Fatigue. 2019;128:105194. doi: 10.1016/j.ijfatigue.2019.105194
- Chernyavsky D, Kononenko DY, Han JH, Kim HJ, Van Den Brink J, Kosiba K. Machine learning for additive manufacturing: Predicting materials characteristics and their uncertainty. Mater Des. 2023;227:111699. doi: 10.1016/j.matdes.2023.111699
- He P, Liu Q, Kruzic JJ, Li X. Machine-learning assisted additive manufacturing of a TiCN reinforced AlSi10Mg composite with tailorable mechanical properties. Mater Lett. 2022;307:131018. doi: 10.1016/j.matlet.2021.131018
- Liu Q, Wu H, Paul MJ, et al. Machine-learning assisted laser powder bed fusion process optimization for AlSi10Mg: New microstructure description indices and fracture mechanisms. Acta Mater. 2020;201:316-328. doi: 10.1016/j.actamat.2020.10.010
- Awd M, Saeed L, Münstermann S, Faes M, Walther F. Mechanistic machine learning for metamaterial fatigue strength design from first principles in additive manufacturing. Mater Des. 2024;241:112889. doi: 10.1016/j.matdes.2024.112889
- Maleki E, Bagherifard S, Guagliano M. Application of artificial intelligence to optimize the process parameters effects on tensile properties of Ti-6Al-4V fabricated by laser powder-bed fusion. Int J Mech Mater Des. 2022;18(1):199-222. doi: 10.1007/s10999-021-09570-w
- Meng L, Zhao J, Lan X, Yang H, Wang Z. Multi-objective optimisation of bio-inspired lightweight sandwich structures based on selective laser melting. Virtual Phys Prototyp. 2020;15(1):106-119. doi: 10.1080/17452759.2019.1692673
- Gong X, Yabansu Y, Collins P, Kalidindi S. Evaluation of Ti-Mn alloys for additive manufacturing using high-throughput experimental assays and gaussian process regression. Materials. 2020;13(20):4641. doi: 10.3390/ma13204641
- Miracle DB, Senkov ON. A critical review of high entropy alloys and related concepts. Acta Mater. 2017;122:448-511. doi: 10.1016/j.actamat.2016.08.081
- Huang W, Martin P, Zhuang HL. Machine-learning phase prediction of high-entropy alloys. Acta Mater. 2019;169:225-236. doi: 10.1016/j.actamat.2019.03.012
- Beniwal D, Ray PK. Learning phase selection and assemblages in High-Entropy Alloys through a stochastic ensemble-averaging model. Comput Mater Sci. 2021;197:110647. doi: 10.1016/j.commatsci.2021.110647
- Krishna YV, Jaiswal UK, Rahul MR. Machine learning approach to predict new multiphase high entropy alloys. Scr Mater. 2021;197:113804. doi: 10.1016/j.scriptamat.2021.113804
- Kaufmann K, Vecchio KS. Searching for high entropy alloys: A machine learning approach. Acta Mater. 2020;198:178-222. doi: 10.1016/j.actamat.2020.07.065
- Choudhury A, Konnur T, Chattopadhyay PP, Pal S. Structure prediction of multi-principal element alloys using ensemble learning. Eng Comput. 2019;37(3):1003-1022. doi: 10.1108/EC-04-2019-0151
- Lee K, Ayyasamy MV, Delsa P, Hartnett TQ, Balachandran PV. Phase classification of multi-principal element alloys via interpretable machine learning. NPJ Comput Mater. 2022;8(1):25. doi: 10.1038/s41524-022-00704-y
- Zhang L, Chen H, Tao X, et al. Machine learning reveals the importance of the formation enthalpy and atom-size difference in forming phases of high entropy alloys. Mater Des. 2020;193:108835. doi: 10.1016/j.matdes.2020.108835
- Dai D, Xu T, Wei X, et al. Using machine learning and feature engineering to characterize limited material datasets of high-entropy alloys. Comput Mater Sci. 2020;175:109618. doi: 10.1016/j.commatsci.2020.109618
- Kim G, Diao H, Lee C, et al. First-principles and machine learning predictions of elasticity in severely lattice-distorted high-entropy alloys with experimental validation. Acta Mater. 2019;181:124-138. doi: 10.1016/j.actamat.2019.09.026
- Pei Z, Yin J, Hawk JA, Alman DE, Gao MC. Machine-learning informed prediction of high-entropy solid solution formation: Beyond the Hume-Rothery rules. NPJ Comput Mater. 2020;6(1):50. doi: 10.1038/s41524-020-0308-7
- Liu S, Kappes BB, Amin-Ahmadi B, Benafan O, Zhang X, Stebner AP. Physics-informed machine learning for composition-process-property design: Shape memory alloy demonstration. Appl Mater Today. 2021;22:100898. doi: 10.1016/j.apmt.2020.100898
- Li Z, Pradeep KG, Deng Y, Raabe D, Tasan CC. Metastable high-entropy dual-phase alloys overcome the strength-ductility trade-off. Nature. 2016;534(7606):227-230. doi: 10.1038/nature17981
- Tapia G, Khairallah S, Matthews M, King WE, Elwany A. Gaussian process-based surrogate modeling framework for process planning in laser powder-bed fusion additive manufacturing of 316L stainless steel. Int J Adv Manuf Technol. 2018;94(9-12):3591-3603. doi: 10.1007/s00170-017-1045-z
- Chen D, Skouras M, Zhu B, Matusik W. Computational discovery of extremal microstructure families. Sci Adv. 2018;4(1):eaao7005. doi: 10.1126/sciadv.aao7005
- Xue T, Wallin TJ, Menguc Y, Adriaenssens S, Chiaramonte M. Machine learning generative models for automatic design of multi-material 3D printed composite solids. Extreme Mech Lett. 2020;41:100992. doi: 10.1016/j.eml.2020.100992
- Fleisch M, Thalhamer A, Meier G, et al. Functional mechanical metamaterial with independently tunable stiffness in the three spatial directions. Mater Today Adv. 2021;11:100155. doi: 10.1016/j.mtadv.2021.100155
- Bessa MA, Glowacki P, Houlder M. Bayesian machine learning in metamaterial design: Fragile becomes supercompressible. Adv Mater. 2019;31(48):1904845. doi: 10.1002/adma.201904845
- Sharma S, Gupta V, Mudgal D, Srivastava V. Predicting biomechanical properties of additively manufactured polydopamine coated poly lactic acid bone plates using deep learning. Eng Appl Artif Intell. 2023;124:106587. doi: 10.1016/j.engappai.2023.106587
- Nasrin T, Pourali M, Pourkamali-Anaraki F, Peterson AM. Active learning for prediction of tensile properties for material extrusion additive manufacturing. Sci Rep. 2023;13(1):11460. doi: 10.1038/s41598-023-38527-6
- Veeman D, Sudharsan S, Surendhar GJ, Shanmugam R, Guo L. Machine learning model for predicting the hardness of additively manufactured acrylonitrile butadiene styrene. Mater Today Commun. 2023;35:106147. doi: 10.1016/j.mtcomm.2023.106147
- Butt J, Mohaghegh V. Combining digital twin and machine learning for the fused filament fabrication process. Metals. 2022;13(1):24. doi: 10.3390/met13010024
- Zhang J, Wang P, Gao RX. Deep learning-based tensile strength prediction in fused deposition modeling. Comput Ind. 2019;107:11-21. doi: 10.1016/j.compind.2019.01.011
- Lee S, Zhang Z, Gu GX. Generative machine learning algorithm for lattice structures with superior mechanical properties. Mater Horiz. 2022;9(3):952-960. doi: 10.1039/D1MH01792F
- He Y, Abdi M, Trindade GF, et al. Exploiting generative design for 3D printing of bacterial biofilm resistant composite devices. Adv Sci. 2021;8(15):2100249. doi: 10.1002/advs.202100249
- Goh GD, Sing SL, Lim YF, et al. Machine learning for 3D printed multi-materials tissue-mimicking anatomical models. Mater Des. 2021;211:110125. doi: 10.1016/j.matdes.2021.110125
- Veerabagu U, Palza H, Quero F. Review: Auxetic polymer-based mechanical metamaterials for biomedical applications. ACS Biomater Sci Eng. 2022;8(7):2798-2824. doi: 10.1021/acsbiomaterials.2c00109
- Sinha P, Mukhopadhyay T. Programmable multi-physical mechanics of mechanical metamaterials. Mater Sci Eng R Rep. 2023;155:100745. doi: 10.1016/j.mser.2023.100745
- Jiao P, Chen T, Xie Y. Self-adaptive mechanical metamaterials (SMM) using shape memory polymers for programmable postbuckling under thermal excitations. Compos Struct. 2021;256:113053. doi: 10.1016/j.compstruct.2020.113053
- Zhang Z, Krushynska AO. Programmable shape-morphing of rose-shaped mechanical metamaterials. APL Mater. 2022;10(8):080701. doi: 10.1063/5.0099323
- Chen Y, Wang L. Periodic co-continuous acoustic metamaterials with overlapping locally resonant and Bragg band gaps. Appl Phys Lett. 2014;105(19):191907. doi: 10.1063/1.4902129
- Belei C, Pommer R, Amancio-Filho ST. Optimization of additive manufacturing for the production of short carbon fiber-reinforced polyamide/Ti-6Al-4V hybrid parts. Mater Des. 2022;219:110776. doi: 10.1016/j.matdes.2022.110776
- Khan ZN, Albalawi HI, Valle-Pérez AU, et al. From 3D printed molds to bioprinted scaffolds: A hybrid material extrusion and vat polymerization bioprinting approach for soft matter constructs. Mater Sci Addit Manuf. 2022;1(1):7. doi: 10.18063/msam.v1i1.7
- Magennis EP, Hook AL, Davies MC, Alexander C, Williams P, Alexander MR. Engineering serendipity: High-throughput discovery of materials that resist bacterial attachment. Acta Biomater. 2016;34:84-92. doi: 10.1016/j.actbio.2015.11.008
- Groll J, Burdick JA, Cho DW, et al. A definition of bioinks and their distinction from biomaterial inks. Biofabrication. 2018;11(1):013001. doi: 10.1088/1758-5090/aaec52
- Chimene D, Lennox KK, Kaunas RR, Gaharwar AK. Advanced bioinks for 3D printing: A materials science perspective. Ann Biomed Eng. 2016;44(6):2090-2102. doi: 10.1007/s10439-016-1638-y
- Zhang Z, Jin Y, Yin J, et al. Evaluation of bioink printability for bioprinting applications. Appl Phys Rev. 2018;5(4):041304. doi: 10.1063/1.5053979
- Qavi I, Halder S, Tan G. Optimization of printability of bioinks with multi-response optimization (MRO) and artificial neural networks (ANN). Prog Addit Manuf. 2024. doi: 10.1007/s40964-024-00828-1
- Lee J, Oh SJ, An SH, Kim WD, Kim SH. Machine learning-based design strategy for 3D printable bioink: Elastic modulus and yield stress determine printability. Biofabrication. 2020;12(3):035018. doi: 10.1088/1758-5090/ab8707
- Xu Y, Sarah R, Habib A, Liu Y, Khoda B. Constraint based Bayesian optimization of bioink precursor: A machine learning framework. Biofabrication. 2024;16(4):045031. doi: 10.1088/1758-5090/ad716e
- Qiao Q, Zhang X, Yan Z, et al. The use of machine learning to predict the effects of cryoprotective agents on the GelMA-based bioinks used in extrusion cryobioprinting. Bio-Des Manuf. 2023;6(4):464-477. doi: 10.1007/s42242-023-00244-4
- Ruberu K, Senadeera M, Rana S, et al. Coupling machine learning with 3D bioprinting to fast track optimisation of extrusion printing. Appl Mater Today. 2021;22:100914. doi: 10.1016/j.apmt.2020.100914
- Hashemi A, Ezati M, Zumberg I, et al. Characterization and optimization of a biomaterial ink aided by machine learning-assisted parameter suggestion. Mater Today Commun. 2024;40:109777. doi: 10.1016/j.mtcomm.2024.109777
- Huang X, Ng WL, Yeong WY. Predicting the number of printed cells during inkjet-based bioprinting process based on droplet velocity profile using machine learning approaches. J Intell Manuf. 2024;35(5):2349-2364. doi: 10.1007/s10845-023-02167-4
- Huang X, Wong YX, Goh GL, Gao X, Lee JM, Yeong WY. Machine learning-driven prediction of gel fraction in conductive gelatin methacryloyl hydrogels. Int J AI Mater Des. 2024;1(2):61-75. doi: 10.36922/ijamd.3807
- Bonatti AF, Vozzi G, Chua CK, Maria CD. A deep learning quality control loop of the extrusion-based bioprinting process. Int J Bioprinting. 2022;8(4):620. doi: 10.18063/ijb.v8i4.620
- Chen B, Dong J, Ruelas M, et al. Artificial intelligence-assisted high-throughput screening of printing conditions of hydrogel architectures for accelerated diabetic wound healing. Adv Funct Mater. 2022;32(38):2201843. doi: 10.1002/adfm.202201843